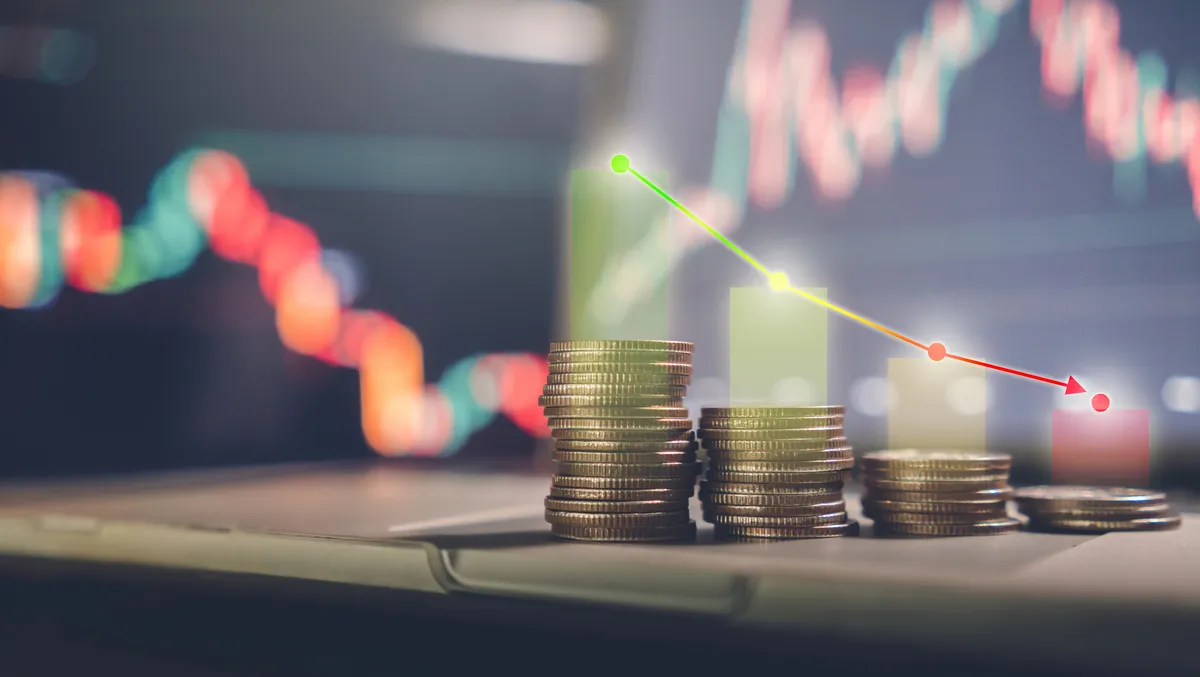
Banks Are Getting to Know You Better - What’s in it for Customers?
Seismic shifts in banking have been experienced in Australia and worldwide due to a mix of new technologies, changing regulatory environments, as well as the response to the global COVID-19 pandemic and natural disasters. The financial sector is experiencing an explosion in data powered by cloud platforms and interoperability. With more data comes the opportunity for increased analytics and insights using artificial intelligence and machine learning to help banks and financial institutions get to know their customers a lot better.
At the recent Australian Retail Credit Association Credit Summit, FICO's Head of Scoring Solutions, Steven Brown, spoke about global credit risk trends. These trends are driving a move to advanced analytics dedicated to making rapid decisions at a customer level and using more transactional data for a better customer view.
Banks will benefit from faster and more accurate decision-making and - ultimately - will be able to deliver a better customer experience.
Focusing on the Customer, not the Account
Traditionally, banks have organised their services at an account level around a single product. Decisions about credit risk have been evaluated through an account lens, rather than the customer's overall portfolio of accounts held with the bank and all the associated details. Account-level decisioning means that every time an existing bank customer applies for a new product - e.g. a line of credit or a mortgage - it is assessed in isolation, even though the bank should already know a lot of details about this customer and their banking history. It's inefficient for the bank and frustrating to the customer.
A shift to customer-level decisions allows for more strategic customer management. Instead of assessing a credit limit increase based solely on credit card behaviour, issuers can consider information from other products held with the bank - such as other credit cards, overdraft limits and loan limits - to determine how much overall exposure they are willing to allow.
Scotiabank, one of Canada's big five banks, recently switched to customer-level decisioning, which has already allowed them to help more customers with more banking services than before. The average number of products per customer jumped from 1.5 to 2.2. The average credit card limit they offer and the number of customers eligible for credit increased by 60 per cent each, as they were able to increase the score cut-off by 40 points, ensuring a lower-risk accepted population.
"The Scotiabank results clearly show there is more room to move with decisions on credit risk when we look at a more holistic view of the customer. The more we understand customers, the better data we have for marketing, for the credit teams, and we can see the story that their transaction data is telling us," says Brown.
How do we turn Data into a Story?
Transactional data needs to be refined before it can tell a story. Transaction scoring refines banking transaction data to make it valuable and useful.
An effective transactional scoring platform combines categorisation profiling, risk score reason codes and income or expense affordability. Rather than the typical monthly summaries used within credit scoring, transaction scoring offers more information, more insights, more 'colour', and more story.
Suppose you compare the monthly summary for two customers, and they look identical. If you look at the daily transactional data for these two customers, it's possible to uncover further dimensions of spending and saving behaviours that were invisible in the monthly summary.
"Shifting from a monthly score to a daily scoring system which incorporates transactional data can help add context to reveal your customer's true position. Are they increasing debt by spending on buy-now-pay-later (BNPL) products? Are they spending enough on non-essentials to show they have a buffer? Perhaps they're showing a high spend on liquor or gambling as a percentage of their total spend, which - in combination with other risk factors or customer details - could potentially indicate added risk," adds Brown.
"These dimensions help paint an accurate picture for more informed decisions on credit risk, propensity, attrition or segmentation and can also be expanded to allow for predictions through artificial intelligence and machine learning models," Brown explained.
Benefits of Daily vs Monthly Risk Scoring
One of FICO's local customers is a major Australian bank that moved from monthly scoring to daily scoring of transactional data across its consumer and small business customers and found significant advantages.
This bank applies decision management using transactional scoring across a FICO platform based on scalable AWS infrastructure.
Using FICO's transaction scoring solution, the bank's almost 20 million consumer retail and SME accounts are now assessed and assigned risk scores on a daily rather than monthly basis. This represents a 3000 per cent increase in scoring data. Of course, that's too much data for human analysis, but with the additional data and daily insights that AI and machine learning provide, the bank has transformed how it makes lending offers, spots defaults and hardship accounts and grows its business.
The new scoring system was combined with traditional models, and the bank has seen an increase of up to 18 per cent in terms of accuracy and outcomes. Over the year, this equated to a substantial increase in its home loan book and small business loan book value. Daily scoring also lets the bank pick up on red flags up to 40 days earlier than monthly scoring, which helps reduce the number of delinquencies and enables a more proactive response during natural disasters.
Potential: Assisting Australians During Natural Disasters
This capability is particularly relevant in Australia and other areas where the recent turbulence in the economic environment due to the pandemic and natural disasters such as fires and floods have put a premium on the ability to be nimble when it comes to lending decisions and customer communication.
FICO's Transactional Scoring solution has the potential to detect deterioration in an individual customer's profile much earlier than a traditional cycle end score would capture. To validate this assumption, FICO used the model to see if this is a leading indicator of hardship events filed due to the 2022 floods in New South Wales using anonymised data from a sample of postcodes that had a high incidence of the natural disaster indicator.
Scores for customers with the natural disaster indicator trended downward, with sharper declines at the start of the flood period. The score trajectory itself may be a predictor of taking up disaster relief. These customers were already trending downwards, and the flood event provided the extra impetus needed to push them into hardship.
The modelling has shown that using this transactional scoring solution, banks could spot this trend 30 to 40 days earlier, allowing them to act sooner and better assist customers. For example, they could reach out with specific offers of extended deadlines or reduced payments on a case-by-case basis, with the goal of assisting customers to manage their loans over time rather than defaulting.
"We're still exploring what's possible with data, particularly transactional data, and it's an exciting time to see the competitive advantages that transactional analytics can deliver our customers," concludes Brown. "All financial institutions - and their customers - can benefit from improved customer communications and the delivery of meaningful and relevant financial products."