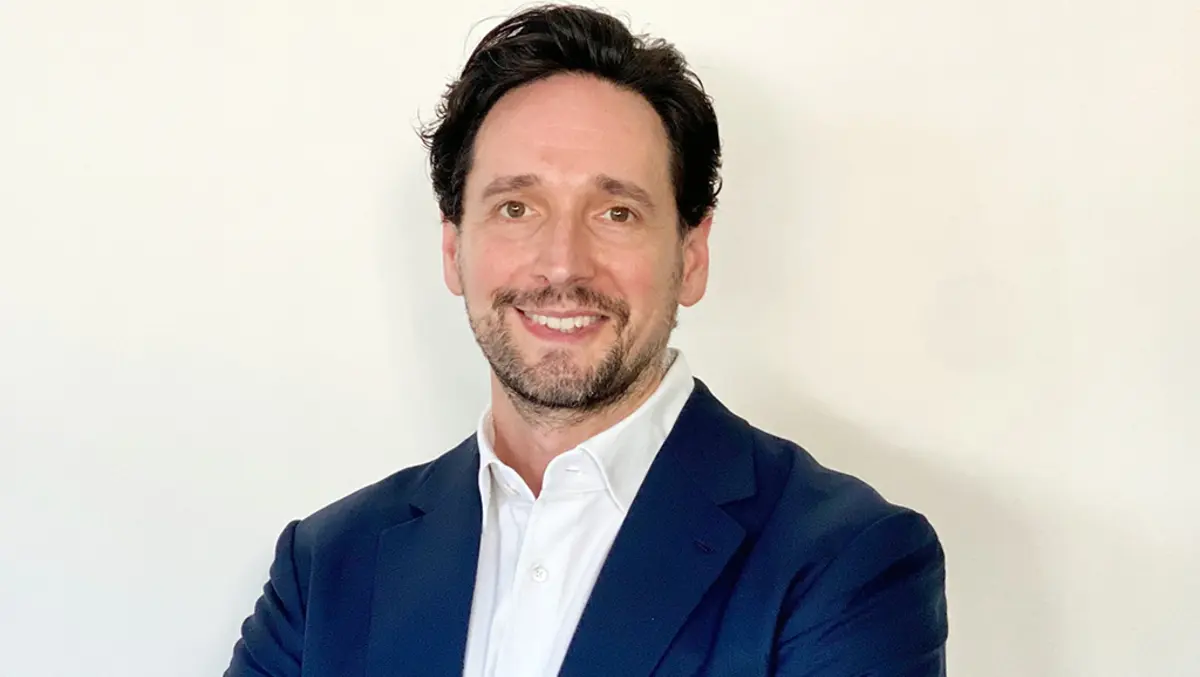
Busting money laundering in Australia is dependent on making better data connections
Two key interrelated themes emerged in AUSTRAC's recent national risk assessment for money laundering in Australia: the persistence of actors engaging in activity and their exploitation of legitimate structures and financial channels to carry out their work.
That second finding won't be a surprise to many.
Over the years, financial crime has been enabled by running the activity in plain sight, masquerading as legitimate and mundane. Funds move through standard financial instruments, transactions, intermediaries, legal entities, and institutions – avoiding detection by banks and law enforcement. AUSTRAC's assessment confirms that this is very much still the case.
"Core features of Australia's domestic economy, such as cash, bank accounts, payments technology, business structures, and trusts, are also used by money launderers to place, layer, and integrate criminal proceeds," AUSTRAC observed.
"Underpinning many money laundering activities in Australia is opacity, anonymity and a lack of transactional visibility. The use of cash, trusts, identity crime, mule accounts and third-party transactions that obscure identity, beneficial ownership or financial flows continues to be a mainstay of money laundering."
But it isn't just the financial sector that's being exploited.
A web of other service providers - including lawyers, accountants, and company trust service providers, and real estate agents - are often engaged to set up legitimate structures that can be used to facilitate money laundering activity. Reforms that would bring these providers under anti-money laundering (AML) rules are being considered, in part to harmonise with efforts internationally.
To be able to run money laundering activities in plain sight requires a level of sophistication to be present. Financial criminals have a lot to play for and are getting better at creating layering techniques and an intricate network of identities and accounts to obscure their activities.
The challenge for banks, service providers, policing agencies, and successive governments alike is to gain better visibility across this ecosystem of financial and business structures. That means becoming even more data-driven than they are today.
Underlying technology shifts
Busting money laundering rings is all about finding connections, and modern technologies are helping to piece these connections together – although some technologies are better than others.
Conventional AML detection measures are aimed at identifying deviations from standard patterns within discrete data and transactions.
At a technical level, they are based on a relational database model where data is stored in predefined tables and columns. While this technology and approach is ideal for many business applications, it does not scale well when applied to the large data sets that piles of financial records often provide. It often struggles to effectively pick up patterns in data that would identify money laundering networks.
The sheer volume, depth and complexity of data needed to pattern-match leads to extensive time being spent investigating and resolving simple, let alone complex, alerts. Large banks commonly process millions of transactions per day that are subject to AML compliance, each of which is associated with one or more parties. In addition, focusing on discrete data alone (so numbers that represent exact values, such as individual transactions without context) also tends to generate many false positives, leading to fruitless investigations.
What is needed is the ability to follow a trail of crumbs from one account to another, tracing the path of a single transaction.
Knowledge graph technology, supported by a graph database, is well-suited for AML work, because it's key aim is to uncover hidden patterns and relationships in data. In simple terms, it focuses on 'nodes' and 'relationships', which means any number of qualitative or quantitative properties can be assigned to describe complex patterns coherently and descriptively. Graph databases place as much emphasis on the relationships between data points as the data itself, meaning that entities such as 'person,' 'account,' 'company,' and 'address,' along with their connections to one another, like 'registered at' or 'transacted with,' then reveal complex connections.
With this richer context, banks can compare a single entity, such as a business or a person, against other similar data entries to identify potential deviations from the norm.
Investigators need to understand the facts and circumstances surrounding a flagged entity or transaction. They must be able to filter, expand paths, and ask ad hoc questions at interactive speeds to explore the full context of the structure, behaviour, and time surrounding the flagged items.
A self-service data visualisation tool that supports real-time, natural language and ad hoc analysis can provide support in this regard, allowing them to assess the risks associated with a particular transaction and eventually build up an accurate picture of the money laundering network.
GraphRAG technology, combined with GenAI that, can help speed up the evaluation of suspicious entities, are taking AML investigations to a new level. GraphRAG adds knowledge graphs to Retrieval Augmented Generation (RAG) and has become essential for addressing 'hallucinations' or inaccurate responses in a form of GenAI known as LLMs (Large Language Models.) It enables organisations to retrieve and query data from external knowledge sources, giving LLMs a logical way to access and leverage their data. Knowledge graphs ground data in facts while harnessing explicit and implicit relationships between the data points, forcing the model to focus on the correct answers. The result makes GenAI outputs accurate, contextually rich, and – critically – explainable."
Real-time analysis that uncovers data patterns is the only effective way to keep one step ahead of the criminal networks and their money movements. Armed with graph database software and AI, the banking and finance sector is taking on the money launderers and winning.