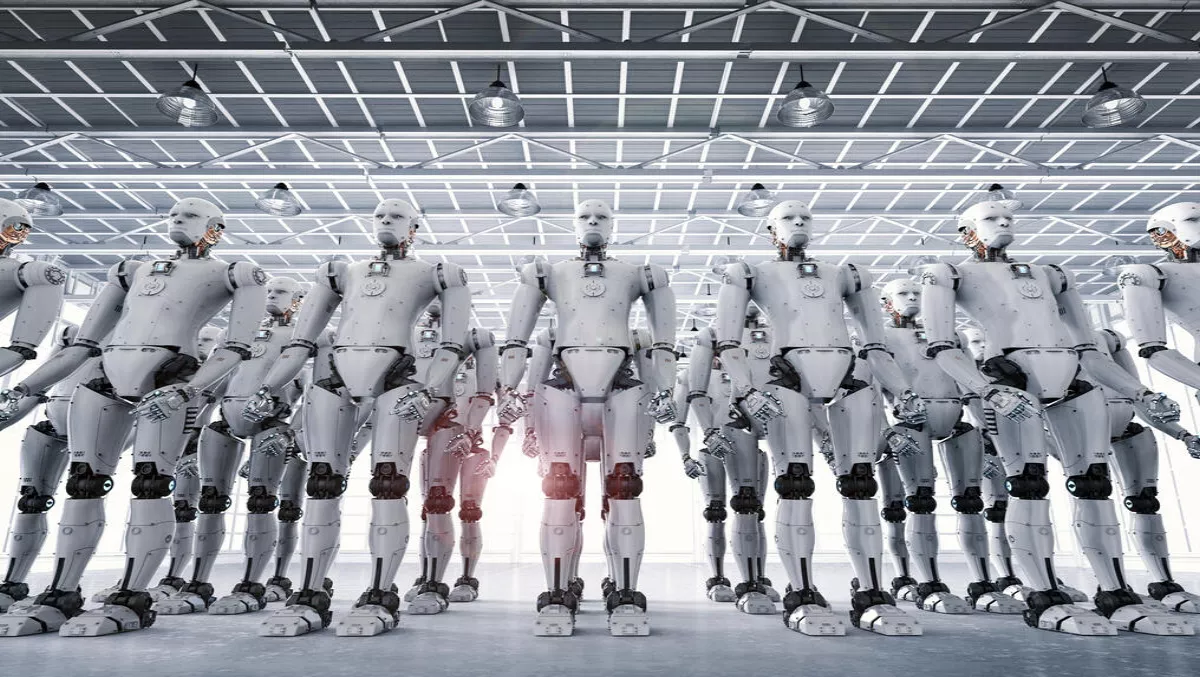
Exclusive interview: What happens when artificial intelligence is racist?
Dr. Rob Walker is the vice president of decision management at Pegasystems, and also an artificial intelligence (AI) expert.
This exclusive interview delves deep into two strands of artificial intelligence - opaque AI and transparent AI. Walker discusses the differences between the two, situations where one is more appropriate than the other, and what happens when we the use of AI becomes unethical.
Opaque AI vs. transparent AI – what's the difference?Opaque AI uses algorithms that are not explainable to humans.
Not just because, in some cases, it's like 'alien' thinking, but also because of the sheer complexity of the resulting (prediction, classification, or decision models).
Examples of opaque models are the multi-layered neural networks used for deep learning, which roughly mimics the inner workings of the human brain; or some of the results of genetic algorithms, a technique that evolves solutions to problems using variants of a simulated survival of the fittest context.
In contrast, transparent AI relies on techniques that can be successfully explained.
Examples are modestly sized scorecards or decision trees that explicitly show how they use data to come to a prediction, classification, or decision.
However, insisting on transparency is a severe constraint on the algorithms used and, as a consequence, opaque AI can be expected to be more powerful, for instance, make better predictions.
Opaque versus transparent is not a delineation between good and bad (after all, human thinking is often quite opaque as well), it's a matter of organisations choosing where understanding trumps performance and where it's the other way around.
Artificial intelligence in the enterpriseThe use of AI in business, especially opaque algorithms like deep learning, is only now starting.
Such algorithms have been in use for a while, but were mostly used in research and applied to cognitive categories involving games (go, poker), recognition (images, speech, emotions), language (translation, generation), robotics (autonomous vehicles, robots), and a few others.
But with the rise of (big) data and far-reaching automation, AI categories like predictive analytics have ventured into the opaque area.
Better predictions are worth a lot of money: approve loans with a lower probability of default, diagnose diseases more accurately, make more relevant offers.
So, the question then becomes, are organisations (and the general public) prepared to trade more transparency for better decisions?
It's safe to say, in general, that the opaque/transparent discussion will be had first in industries that are heavily regulated.
For instance, a bank that could potentially use (opaque) deep learning to better select those applicants that are likely to repay a loan (plus interest), may find themselves struggling explaining their risk exposure.
After all, their opaque algorithms may be more effective, but they can't explain why certain applicants are accepted and others rejected.
If an opaque algorithm tells a bank that customer X is a 0.9 possibility of getting into default that's one thing; if it can't explain why, that's a very different thing.
The dark side of AIWithin the scope of customer engagement and CRM, the dark side of AI would manifest itself by making decisions (to yes/no grant a loan, make a certain offer, increase a priority, invest in retention) using sensitive customer traits (gender, religion, race, age, etc.) without explaining that to human supervisors.
Therefore, customer strategies can go into effect with profound negative, perhaps even illegal, consequences.
Because opaque AI, even when it's better at its task, cannot explain why or how it comes to a decision, the risk is that those decisions do not comply with legislation or even corporate policies.
What if an algorithm is racist?
In an opaque algorithm, it may be hard to find that bias by inspecting the model. A complicated factor is that modern algorithms (opaque and transparent) can easily make undesirable inferences from the vast amount of (big) data they are given.
In other words, to be racist, an AI doesn't require a customer's race to be held in any database.
It can infer race (or gender, age, sexual orientation, intelligence, mental health, etc.) through innocent looking attributes and subsequently use it for a selection or decision.
If the algorithm inferring any of those traits is opaque to boot, it's impossible to know how it's using them to come to a decision. It's a decision made by a black box that can't explain itself.
Now, even though that sounds very undesirable, humans are often black boxes as well.
They will use words like intuition or gut feel to describe opaque thinking, or, more often, are not even aware their ideas or insights are not 'logical'.
So – which is better?Obvious scenarios where opacity should be considered more than just carefully are those in which AI make decisions (or even just recommendations) where such decisions have a meaningful impact, especially if they're incorrect.
Sending someone an irrelevant offer may not be the end of the world, but incorrectly rejecting a mortgage or job application is something where a black box is likely not acceptable.
In the EU, the General Data Protection Regulation (GDPR) will got into effect in May 2018, affecting not just EU companies but any company with EU customers.
Among many other things, GDPR requires an explanation for any automated decision that has 'legal significance'.
Lawsuits will soon define the legal boundaries of 'significance' but it's a safe bet that under GDPR, opaque algorithms are a liability where such decisions are concerned.
The balance between machine and human decision making in the enterpriseTools like Decision Management support very explicit collaboration between AI and human decision making.
The AI provides, either through transparent algorithms, opaque algorithms, or a mix, higher order input for a human decision maker.
For instance, the AI would determine whether a marketing offer is relevant, but a human can decide how much irrelevance is acceptable to the brand; are they going to spam a lot of prospects to harvest a higher number of responders, or is relevance important to them so that their customers continue to read their messages and feel the company knows them?
Alternatively, AI takes over all of this and maximises ROMI (return on marketing investment), but humans responsible for the brand promise will decide whether selling is appropriate in the first place, versus risk management, retention, or service.
These are examples at the core of the organisation, but in the front-office AI will often recommend a course of action to a human agent.
However, one should not be naïve about such collaboration.
If the AI is clearly superior, human agents will be expected to simply do as recommended (or just do it to make their targets); if the AI is not, it will be ignored.
In the front-office humans are probably still marginally better at detecting emotions or non-obvious intents.
While that's still the case, collaboration between artificial and original intelligence may still beat either.
And of course, not every human is comfortable with chatting with or talking to something evidently artificial; or, if not evident, feel tricked into thinking they're conversing with a human agent.
In the near future, that distinction will become hard to make while at the same time customers will likely become more tolerant, especially if an AI delivers superior advice, has full context, and is more patient.
The best collaboration is where AI and human judgment each play to their own strengths.
AI is undisputedly better in finding complex patterns in vast amounts of data and thus often supplies higher quality probabilities and predictions.
Humans can take that automated insight and apply strategy. That boundary, too, will shift over time, and shift fast, but for now it's a workable and effective balance.
Unchecked AI: The ethical sign-offOpaque AI is less safe than transparent AI because it's a black box.
Its decisions, meanwhile, can be superior. Therefore, it's not a straightforward matter of banning opaque algorithms.
Superior decisions, even by a black box, can increase profits, reduce risk, or save lives (in a clinical setting, for instance).
What's required are two things:
1) a setting in any decisioning system relying on AI to restrict the use of opaque algorithms to areas of the business and to use cases where an inability to explain is not critical.
And 2), where allowed, to test for 'rogue' biases in the decisions made.
Because there's no way, in opaque AI, to reverse-engineer the internal decision-making process, we can only judge the black box by what it does (versus how it does it).
That's where the ethical sign-off comes into play. The AI is asked to make decisions about a selection of customers/prospects for who certain sensitive traits are known (gender, age, etc.) and those decisions, rather than the underlying algorithm, are then analyzed for undesirable biases.