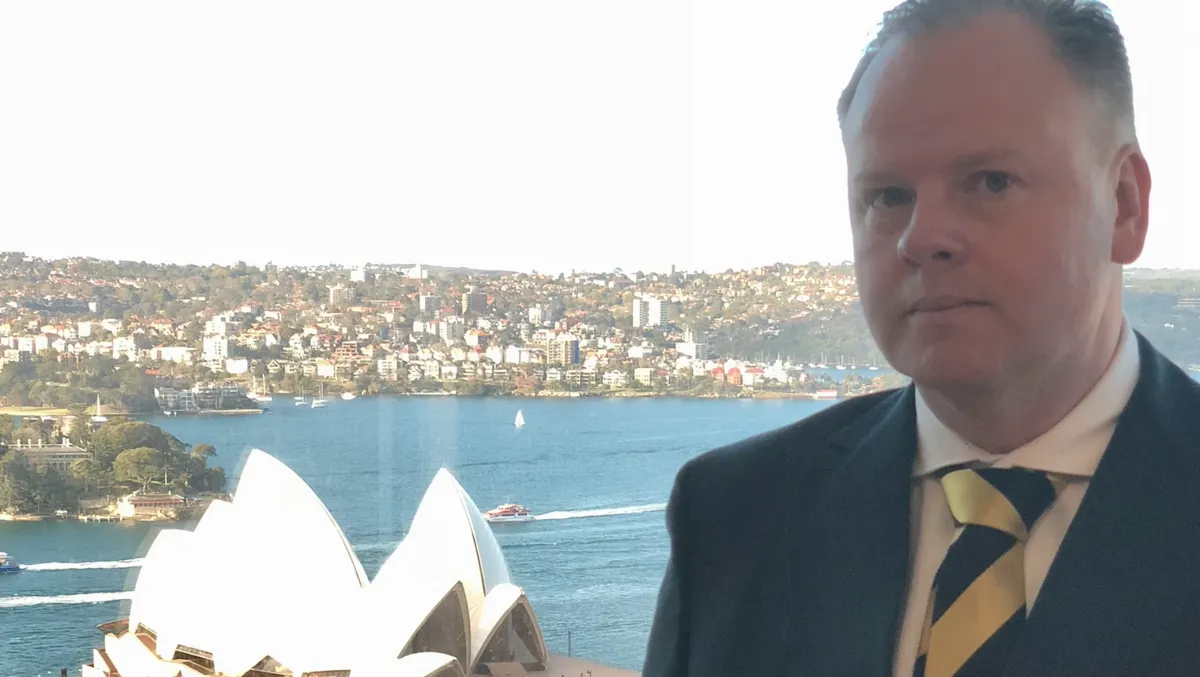
Five key discoveries enterprises have made in their AI journeys so far
As we approach the first year since AI well and truly burst into the enterprise and mainstream consciousness, it's worth reflecting on what the year has been like for early adopters.
While many have experienced promising early results, it is often in non-critical scenarios. The utility value is promising, but the tools are often not being used on core or critical business problems.
In addition, the more enterprises learn about AI and its practical applications, the more it often leads to a change in the strategic direction and approach to adoption.
In our experience, enterprises have made five key discoveries in the past year of experimentation.
Discovery #1: Experiments may produce unrepresentative results
For many enterprises, their introduction to AI has been through ChatGPT, a conversational interface for the GPT large language model (LLM). What many have come to realise through their experiments is that LLMs on their own are not necessarily representative of AI's power or potential within enterprise environments.
In contrast, Conversational AI platforms create end-to-end dialogue experiences. Virtual agents powered by Conversational AI are capable of handling complex conversations with customers and staff and dealing with multiple twists and turns in that interaction. An LLM may play a role as an individual piece of the end-to-end chain, but other pieces are also required. When enterprises realise their early experiments with LLMs fall short of the broader construct, results, and value that a Conversational AI platform can and should generate, it generally leads to a reconsideration and broadening of their AI strategies and approach.
Discovery #2: Unpredictability is hard to productionise
The unpredictable nature of how LLM-powered applications arrive at a response - and the extent to which they can be goaded into making false statements or reaching demonstrably wrong conclusions - poses a high risk to would-be enterprise adopters. While Australian enterprises have a reputation for early adoption of emerging technology, even their risk appetite can only be stretched so far. Standalone LLMs in a critical production setting - such as an automated dispensary of financial advice offered to bank customers - could be prompted to make false or misleading statements about a customer's financial status or position, recommend poor investments or understate risks. The potential for this to cause real-world impacts to the customer and 'bad headlines' for the enterprise cannot be ignored.
An enterprise-ready approach to AI is one that covers the end-to-end technical architecture and where usage is auditable, repeatable, and predictable. Every time a user asks a certain question, enterprises want to know that a certain response and process is invoked that will give the questioner an acceptable answer and outcome. Consistency establishes a corporate comfort level with the technology.
Discovery #3: Every organisation's AI journey is different
An organisation is the sum of years of investments and decisions. Each has evolved differently, with its own combination and configuration of technology systems that drive processes and enable it to operate. Through early experiments, many organisations have reached the same conclusion: that it's not trivial to assemble the components of a Conversational AI that can interface with some or all of these different customised systems. It is hard to find an out-of-the-box solution that can deal with the variation.
For this reason, choosing the right Conversational AI platform and partner is an important decision. An ideal technology partner and platform should be able to start wherever the customer needs it to be. It may also be backed up by a maturity model approach, where customers can self-select the pieces they implement based on where they are in their AI journey. Their needs on day one, while they're still learning about the technology, will be vastly different to when they have a virtual agent in production and want to mature the capability to optimise its performance, benefits, and value delivery.
Discovery #4: AI needs to be integrated with other systems to really show its value
Conversational AI can be powerful in isolation if it understands what a user is saying and returns a contextual text-based response. However, this can only achieve so much. The power of Conversational AI is multiplied when the AI-generated response drives a relevant and personalised transactional experience that ultimately helps that user save time. That means having a Conversational AI engine that is integrated with other enterprise systems so it can complete tasks on behalf of customers and staff.
For example, a person wanting to book a doctor's appointment will have a better experience with a virtual agent if the AI both understands their need and uses an integration to the medical appointment booking system to schedule an appointment. Or, an employee who wants to book annual leave will view a virtual agent more favourably if it listens to them and gets their leave booked into the appropriate HR system.
Discovery #5: It's time to show some ROI
Enterprises have put, at times, considerable effort into developing Conversational AI applications and use cases. For that expenditure of effort and resourcing commitment to continue, many want to see a bigger return on investment, as well as reduced time-to-value.
Out-of-the-box solutions can help enterprises achieve these goals. If an enterprise can start with half of the solution pre-built for them, their time-to-value is drastically reduced. If a medical practice wants to enable bookings via a virtual agent, ideally, they should be able to write a single prompt and have their platform build out the conversational flows, intent and entity models and integration placeholders that are required. This would allow the enterprise to get a working prototype together in minutes, decreasing time-to-value, time-to-market and overall TCO. It also means enterprises can create use cases that generate material revenue or savings - exponentially increasing the overall value they get from being in the Conversational AI space.