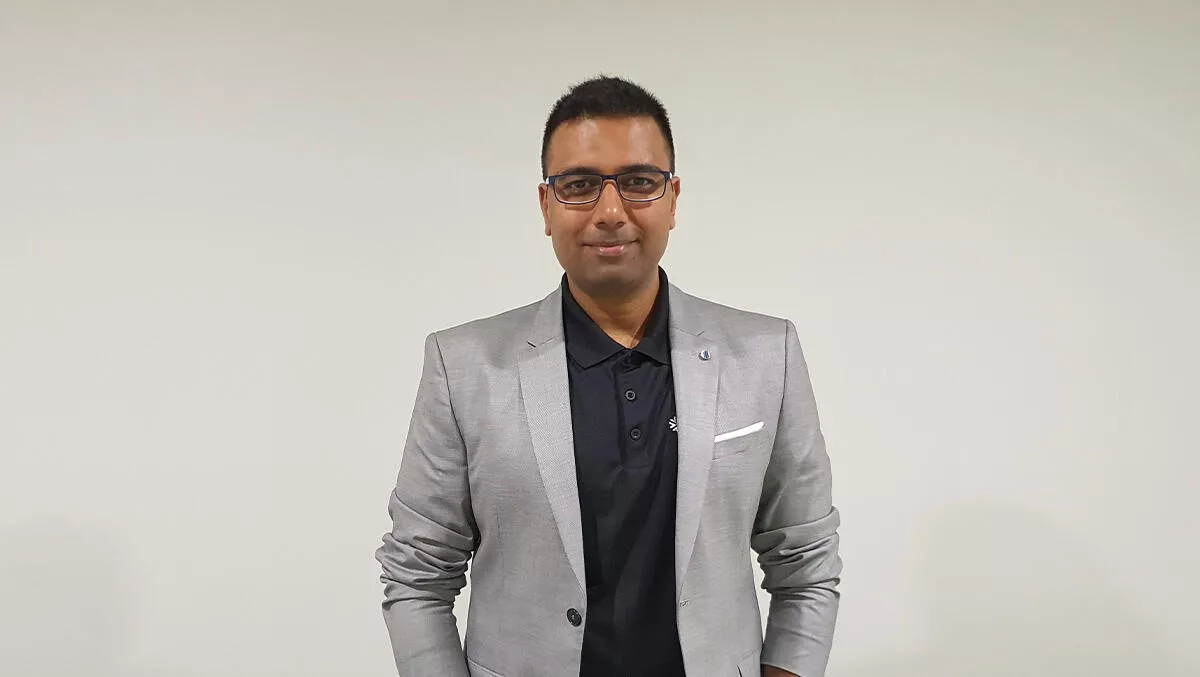
Four factors to consider before using AI to augment data analytics
As a rapidly developing technology, artificial intelligence appears to have much to offer organisations battling with a rising tide of data.
While traditional approaches to analytics limit the size of the data sets that can be analysed, adding AI means those limits can be significantly increased. This shift opens up new opportunities to gain better insights and deliver significant business benefits.
With this in mind, four key factors need to be considered when determining when and how AI and machine learning tools should be used to augment traditional statistical analysis.
The size of the data set
When it comes to analytics, the most important variable is the size of the data set. When it gets beyond a certain number of rows and columns, traditional statistical tools quickly run out of capacity to identify all the correlations and understand what's interesting or important.
AI tools can augment traditional approaches by allowing staff to analyse more data and identify insights or relationships that would otherwise remain hidden. These tools can also increase awareness that the available data sets do not provide enough information for accurate predictions.
Working with larger data sets ensures higher accuracies on a wide range of algorithms, which these days are only a click away.
Explainability versus performance
Another consideration is the level of 'explainability' required for problems. For example, if you're learning to control a self-driving car, you don't need to understand the impact of every variable on the output. You only need a high degree of confidence that it is safe.
However, if you are predicting whether a piece of machinery will fail, you need to understand the impact of each variable, including temperature, humidity, and the rotation rate of the machine. If not, the output won't be useful because it doesn't allow you to take corrective action.
Explainability is important in other contexts, too. If a model produces results that align with intuition, then the model you spent time building may not be used. For instance, if a model predicts that a certain change will increase sales by 40%, but you can't explain why, people may reject it because they don't trust the result.
Selecting the right algorithm
Different algorithms have different advantages and disadvantages. For example, in forecasting, you might choose between ARIMA, ARMA, Prophet, or LSTM. It's recommended to consider which are best supported by the broader community and the feature differences between them. Other questions to consider include their limitations and what biases they might inherently introduce.
These are nuanced considerations that are not equally weighted and will depend on the business or analytical problem at hand. Working with domain experts and carefully considering the use case will be critical to choosing the most appropriate algorithm.
Mapping domain knowledge to the problem space is also an important factor when selecting the right algorithms. For example, you may find that there are multiple algorithms to handle classification. However, classification is a diverse area, and what constitutes the right algorithm may vary depending on the nuance of an industry or use case.
Achieving execution velocity
For most organisations, true value is found in the data and not in tuning the algorithm so that practitioners can accelerate their use of machine learning with AutoML tools. These tools allow teams to test 20 or 30 algorithms against the same data and compare the performance of those models. That allows for a level of iteration and visibility not previously possible, and it's a powerful way to test classic algorithms in a much more expansive way.
However, there are trade-offs here too. If five models produce similarly high-performance scores, how do you decide which one is preferable? One option is to conduct A/B tests using a subset of the data to gather empirical evidence about which model truly has the best performance. This is a good general MLOps practice, both when a model is first created and whenever a model is updated.
Adding business value
By adding AI and ML capabilities to a data analyst's toolbox, a business can extract significantly more value from its data than is currently the case. However, several key factors need to be considered.
Taking the time before rolling out these tools to understand their potential (and limitations) will ensure expected benefits are achieved.