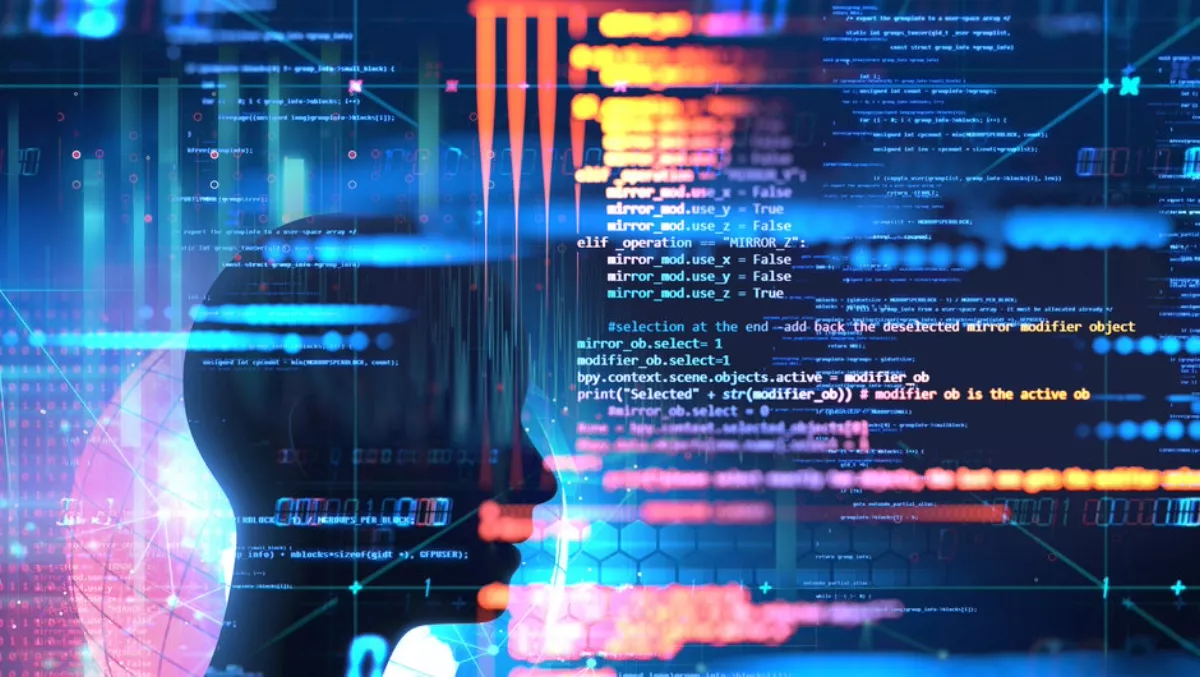
Fujitsu develops automatic labelling technology to accelerate AI
Fujitsu Laboratories and Kumamoto University have announced the development of technology to easily create the training data necessary to apply AI to time-series data, such as those from accelerometers and gyroscopic sensors.
Time-series data obtained from sensors do not include anything other than ever-changing numerical data. Therefore, in order to create training data for use in machine learning, it was necessary to manually attach finely detailed labels to the data in accordance to its changing values, indicating what was done and when at each point where the numerical values changed. For this reason, huge numbers of man-hours were required, and the use of AI with time-series data had seen limited progress.
Fujitsu Laboratories and Kumamoto University have supposedly enabled the automatic creation of highly accurate training data with appropriate labels for each action, just by manually attaching a single label to each longer time period, even if they include multiple actions, indicating the major action in that time period according to human judgement.
Because this significantly reduces the number of man-hours required, this technology will accelerate the use of AI with time-series data. The new development is expected to enable easier installation of services such as fall detection, operational functionality checks, and abnormality detection for machines, in smartphones and various other devices.
Development Background
In recent years, with the evolution of technology such as the Internet of Things (IoT), it has become possible to obtain a large amount of time-series data from a variety of sensors. For example, by developing functionality in which AI can determine the meaning of the motions of people and objects from the characteristics captured by accelerometers, it is expected that advanced functionality for monitoring people and machines can be incorporated into smartphones and various equipment. In order to apply AI to this sort of time-series data, it is necessary to create training data to train AI.
Effects
Fujitsu Laboratories and Kumamoto University conducted a trial where they attached labels to time-series data from accelerometers while performing mock work processes in a factory such as polishing. As a result, they confirmed that this technology was able to correctly label 92% of time periods.
They judged that this was equivalent to the highly accurate results obtained when using data that was manually labelled in detail as training data. With this technology, one can easily create training data for AI use from time-series data, and further development is expected for the functionality that uses AI to determine activities captured by sensors.
In addition, because this technology makes determinations based on just the numerical characteristics of time-series data, and does not rely on the type of sensor, it could also be applied to time-series data obtained from devices such as temperature sensors and pulse wave sensors.