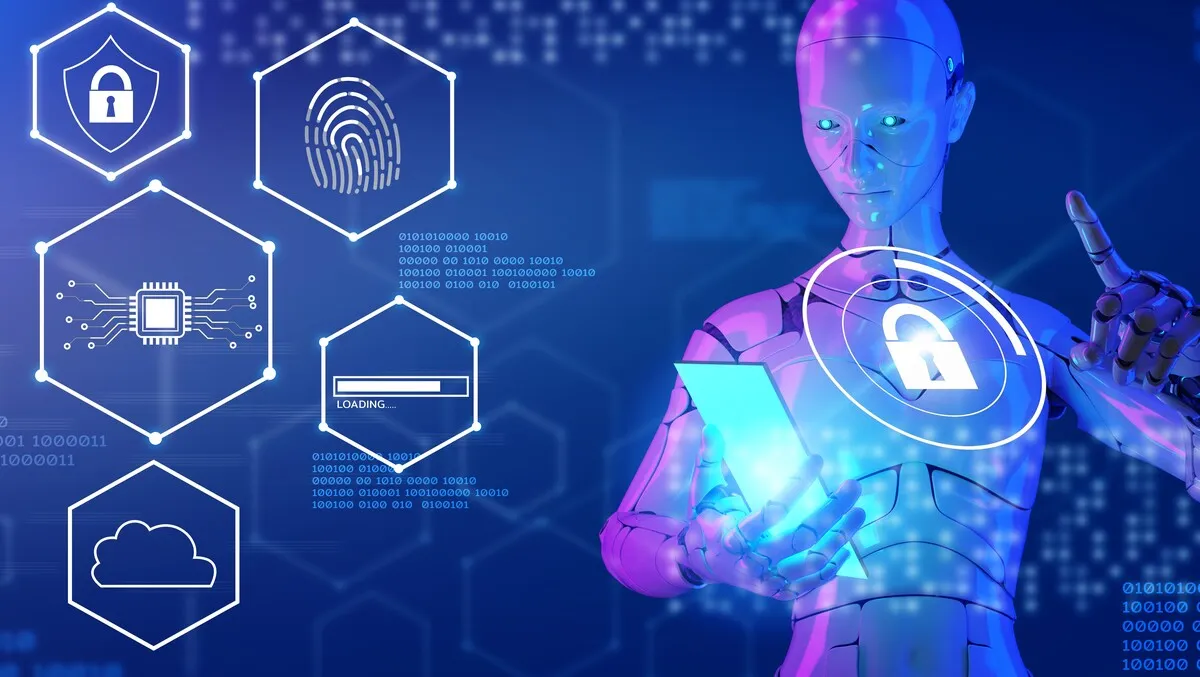
The advent of Artificial Intelligence (AI) has brought about revolutionary changes in virtually every sector of society. However, the promising capabilities of AI are accompanied by significant challenges, particularly in cybersecurity.
At Mantel Group, we’ve been helping large organisations with their security challenges since our inception, with projects ranging from advisory and vulnerability remediation, cloud hardening, DevSecOps and compliance. Securing Machine Learning workflows has become essential to protect these organisations from emerging threats, and one of those emerging threats is adversarial AI.
What is Adversarial AI?
In simple terms, adversarial AI refers to a set of techniques used to deceive AI systems. By exploiting vulnerabilities in the AI models, adversaries can trick these systems into making erroneous decisions that favour the attacker’s motives. These techniques have become alarmingly prevalent in recent times. In 2022 alone, 30% of all AI cybersecurity incidents utilised adversarial techniques, according to a survey conducted by Microsoft. Despite the danger, most organisations are woefully underprepared, with Microsoft finding that roughly 90% of companies lack any strategies to account for Adversarial AI attacks. This is particularly worrying considering that every adversarial AI-related attack has the potential to be classified as a ‘Critical’ incident to an organisation’s cybersecurity, causing substantial damage to both reputation and revenue.
Current Gaps in Cybersecurity Solutions
Despite advancements in cybersecurity solutions, there remain substantial gaps that leave organisations vulnerable to adversarial AI. Cybersecurity has traditionally been focused on protecting networks, devices, and software applications from threats. However, AI brings in a new dimension to cybersecurity, necessitating novel approaches to defence. Many existing solutions overlook AI-specific considerations, including Authentication, Separation of Duty, Input Validation and Denial of Service Mitigation. Without addressing these concerns, AI/ML services are likely to remain vulnerable to adversaries of varying skill levels, from novice hackers to state-sponsored actors.
Overcoming the Challenges: Key Elements of Secure AI
To create robust defences against adversarial AI, we must weave security into the fabric of our AI systems. Here are four key elements to consider:
- Bias Identification: AI systems should be designed to identify biases in data and models without being influenced by these biases in their decision-making process. Achieving this requires continuous learning and updating of the system’s understanding of biases, stereotypes, and cultural constructs. By identifying and mitigating bias, we can protect AI systems from social engineering attacks and dataset tampering that exploit these biases.
- Malicious Input Identification: One common adversarial AI strategy is to introduce maliciously crafted inputs designed to lead the AI system astray. Machine learning algorithms must therefore be equipped to distinguish between malicious inputs and benign ‘Black Swan’ events, rejecting training data that has a negative impact on results.
- ML Forensic Capabilities: Transparency and accountability are cornerstones of ethical AI. To this end, AI systems should have built-in forensic capabilities to provide users with clear insights into the AI’s decision-making process. These capabilities serve as a form of ‘AI intrusion detection’, allowing us to trace back the exact point in time a classifier made a decision, what data influenced it, and whether or not it was trustworthy.
- Sensitive Data Protection: AI systems often need access to large amounts of data, some of which can be sensitive. AI should be designed to recognise and protect sensitive information, even when humans might fail to realise its sensitivity.
Importantly, securing ML models against adversarial AI is not a one-off task but a continuous process that spans across the entire lifecycle of the ML model – from development to deployment and during an attack.
At Mantel Group, we understand the nuances of AI and Cybersecurity. We can assist your company in each of these areas, helping you become more resilient to adversarial AI attacks. Our custom solutions are designed to address your specific needs, helping you become more resilient to adversarial AI attacks. We believe in building AI systems that are not just smart but also secure.
Securing machine learning workflows against adversarial AI is more than a necessity; it’s an obligation to safeguard businesses and customers. By incorporating these elements into your AI systems, you can build a strong foundation for securing AI against adversarial threats.