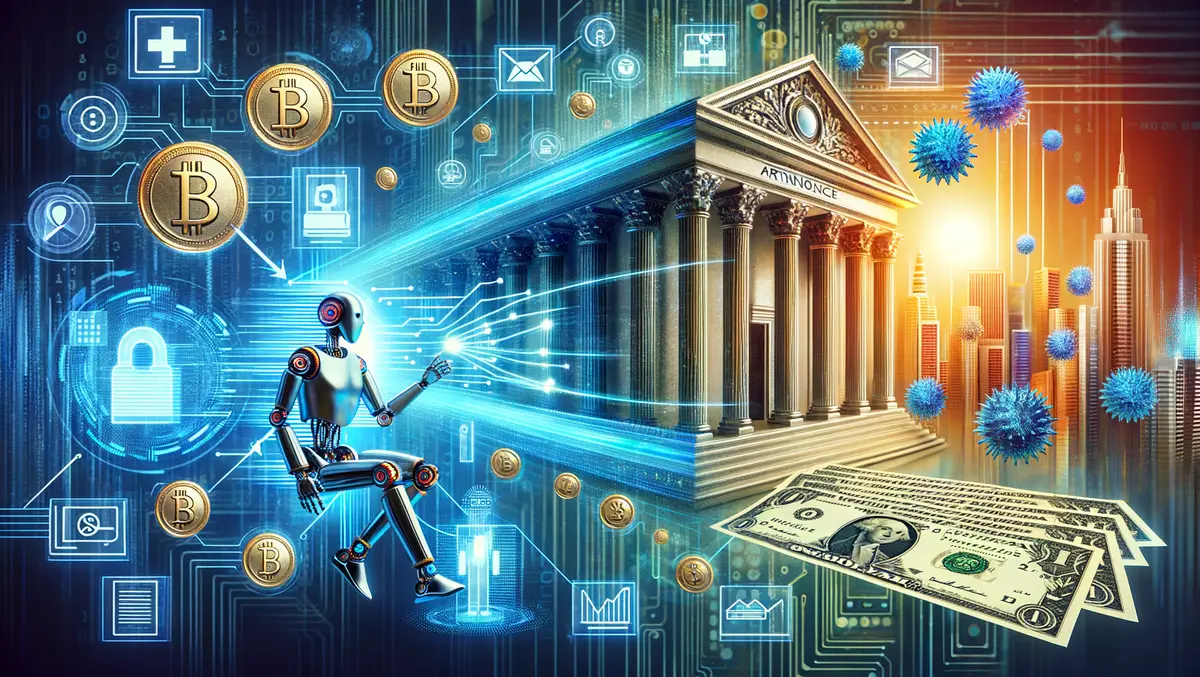
The finance industry has arguably been the most transformative in terms of the impact of technology. Some 20 years ago, trading was an area of bartering over the phone using personality and persuasion, but today is almost entirely automated on supercomputers. And, while there are still hedge fund managers making a living, automated investing platforms like Vanguard are taking their customers at an unprecedented rate.
The same transformation is happening in lending. The days of explaining your business plan to a bank clerk are coming to an end, as it’s quickly being replaced by form-filling, document-scanning AI. if you have a question, though, you can ask its LLM chatbot that replies within two seconds…
Technology can be said to improve lending in five key ways: Automation, accessibility, customer experience, security, and personalisation. Below is a deep dive into the implications of that, both on customers and potentially society as a whole.
The technology driving lending
Most immediately, AI has improved the operational efficiency of lenders. Everything from customer onboarding to underwriting has become streamlined, and this can reflect in better rates for customers, because companies have cut costs in other areas.
While “Artificial Intelligence” is a legitimate computer science technique, AI has been overused as a marketing term. This happens in every industry, whether it’s IPA in craft beer or Organic labels within agriculture. Eventually, they transcend the science into being a branding tool.
What we do know, however, is that AI is incredibly proficient at analysing large amounts of data, along with generating text and predicting outcomes. Figuring out which claims of AI usage are legitimate requires knowledge of the technology, but when it comes to lending, branding tricks are irrelevant. One way or another, customers just want approval and funding.
But, sometimes the creators of an AI tool cannot verify exactly how the model is working, in part because of black-box obfuscation. They know the inputs and outputs, but not the path it took to get there. One example of this is Youtube’s feed, in which Alphabet cannot account for why you’re recommending some videos and not others.
A financial example of this is regarding HMRC, the UK’s tax collection department, which uses 55 billion items of taxpayer data to find patterns. The system ‘Connect’ uses machine learning on 6100 gigabytes of data to identify tax evasion, but this data can reach as far as social media (i.e. common social circles on social media that partake in tax evasion), flight sales, email records, UK Border Agency, and much more.
Within lending, the use of AI can range from being a cost-cutting measure to sifting through rule-breaking behavior, such as Tiimely’s “lending by exception rule”, where the platform flags applicants that have broken any of the 136 rules.
However, more elusive AI decision-making also exists within lending, such as with Zest Automated Machine Learning (ZAML), which is used to inform lenders to make better credit decisions. This model uses Big Data from thousands of variables that traditional credit scoring overlooks. However, due to the stringent regulations within the industry, a lot of effort has gone into making such systems transparent and explainable. But, regardless of how the technology works, it speaks for itself when it comes to the accuracy of predictions.
Speedy, performance-based lending
When issuing a loan to a small business, innovative lenders are using bank transactions to be automatically scanned and help determine the company’s performance. While credit scores are checked very quickly, they never provide enough context, meaning that their immediate accessibility couldn’t be reflected in a fast approval process. With AI algorithms churning through statements and invoices, there’s enough context to make definitive decisions, and unsurprisingly, these can be done in seconds.
It’s also opened up the door to more alternative lending approaches too. While some businesses may not warrant a large loan due to an upcoming off-season, other arrangements make much more sense, such as selling a portion of future invoices through invoice factoring or merchant cash advances. The latter also uses technology to automate the repayment process, in which lenders can be assured that the company will make repayments so long as they have sales, rather than relying on them to responsibly set aside these funds to make manual repayments.
Open Banking and Interoperability
Technology has also paved the way for better interoperability between platforms too, meaning that a better sharing of resources can improve transparency, fairness and speed of decisions. The best example of this is Open Banking, which was brought about by the UK and European Union’s regulation (but has since expanded).
Open Banking allows third parties to access financial data through APIs, meaning that data can be standardised, secure, regulated, and shared easily. For example, a regulated personal finance app can access certain information from all your bank accounts (if the user authorises this), analyse spending patterns through various algorithms, and make suggestions.
The same goes for delivering lending products more efficiently, such as merchant cash advances, where open banking is a key part of the application process where lenders can view the applicant’s banking data.
Its directness isn’t only faster, but it allows for a one-size-fits-all machine learning approach where applicants can quickly plug in their account data. This can even eradicate the form-filling nature of applications, too, as we move towards access tokens (i.e. log-in with Google). The scope for improving financial information relevant to lending applications is vast.
Will AI decrease the wealth gap?
Perhaps the biggest trend within lending has been a movement away from credit scores, a practice that is now seen as 2-dimensional and lacking in context. While it is very much still a part of the regulatory furniture, as it’s illegal to grant a bank loan without a credit check in the UK, it’s becoming relegated to just a minimum requirement, or even a compliance hurdle, while other variables play a bigger role in the terms, size, and APR of the financing.
This is perhaps why merchant cash advances and other alternative lending practices are growing, because they do not legally require a credit check. This isn’t just a benefit for the borrower, but the lender too.
Joe Fainton, a consultant for the Australian financing search engine MNY, puts forward the argument that AI can help mitigate wealth gaps. Once you have bad credit, perhaps due to past mistakes or being illiterate on the gamification of credit scoring, it becomes increasingly difficult to borrow - even years later.
With AI and better technology, it becomes easier to understand the underlying reasons for previous non-payments and banks and lenders will get a more holistic view of every potential borrower. It’s not only more meritocratic, where recent cash flow, decisions, and “performance” is what is rewarded, but it’s more informationally equitable.
Information inequality
Asymmetric information is one of the largest disruptors of many financial services, such as insurance. In lending, it creates a problem that borrowers do not know how to solve. There are guides on improving one’s credit score, but they’re best guesses and approximates.
For example, many people aren’t aware of the importance of credit utilisation, meaning they borrow 80% of their credit limit on their card with the intention of a full and immediate repayment, yet get punished for having a high ratio.
It isn’t fair to expect everyone to know all of the rules of the game they’re playing, because the game has hundreds of rules and they’re not clearly defined. It’s also highly concerning that our skin color could also be a rule…
The implications of ditching credit scores
The implications of our credit scores reach far beyond lending. With studies finding correlations between our cardiovascular health and credit score, it’s become a tool to determine high-risk activity and a way to judge customers within car insurance, utility firms, and even employers. While this raises a lot of privacy concerns, it’s a clear condemnation of those with poor financial literacy, which is already a symptom of poor education, poverty, and single-parent households.
In other words, credit scores have become a perpetuator of inequality in all areas of life. It’s also a perpetrator in a very different way, too, as poor credit scores are a big reason for killing the entrepreneurial spirit within people. Many do not even attempt to start businesses when they know they have a poor credit score, because early financing is an important part of scaling up a business.
When comparing this to the newer model of judging recent performance within a business, a profitable early company (some alternative lending is possible for businesses with 3 months trading history or less) can prove itself very quickly into gaining funding. The skin color, upbringing, and past decisions no longer matter if the algorithm approves of the past three months of bank statements.
Final word: The dangers of AI
It’s not guaranteed that AI will take this direction. As we know, it can process so many seemingly irrelevant inputs into a network too complex for us to understand, and produce results, a little bit like ZAML. This itself has its own concerns that are similar to credit scoring, and in fact, could be potentially much more discriminatory.
This is where regulation and oversight are needed for the algorithms used by companies, where lenders must be able to account for their approvals and rejections. But, it’s also the choice for customers to ditch the practices of ZAML or credit scoring, and head to companies that are more meritocratic and transparent.