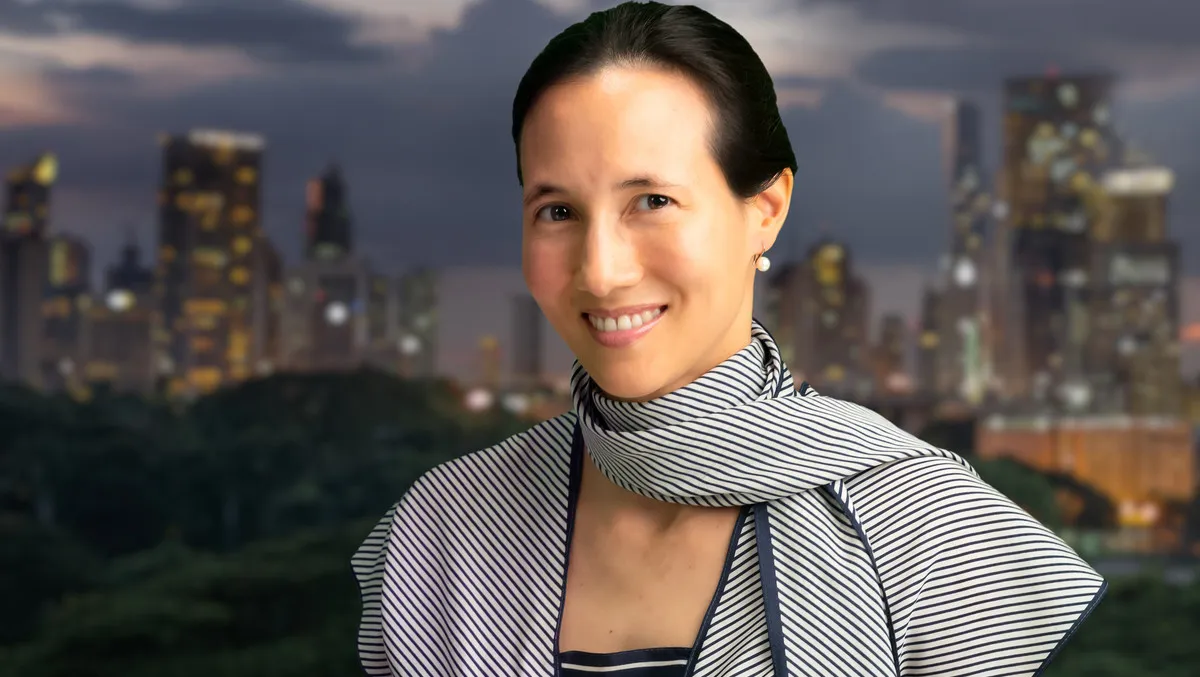
IWD 2023: How organizations can reduce gender bias with Data Science and AI
In the past decade, Southeast Asia has seen tremendous technological transformation enabled by the region's growth in digital consumers. The financial services market, in particular, has seen more consumers moving from traditional banking in physical branches to accessing banking through mobile devices. McKinsey Global Institute reveals that consumers in Asia actively using digital banking jumped up to 88% in 2021, compared to a mere 68% four years ago.
Despite the immense growth the region has seen in recent years, a majority of consumers continue to be unbanked or underbanked. Furthermore, research has shown that the consumer lending market process is stacked against certain groups of applicants—women and minorities, where women are 15% less likely to be approved than men with the exact same credit profile. Many question whether this is due to biases or a statistical anomaly.
While artificial intelligence (AI) presents an opportunity to transform how we allocate credit and risk, creating fairer and more inclusive systems, many have concerns that AI will do just the opposite in this situation. Studies suggest that gender disparity is happening because data is not primarily used to the benefit of financial inclusion and fairness in most cases.
The rise of fintech and algorithmic decision-making has pushed concerns to an all-time high. However, if historical data has led to biased outcomes driven by humans in the past, then the question remains — won't AI-driven lending decisions only amplify biases?
Examining the impact of gender data on creditworthiness
Many may argue that by simply taking out gender information when assessing creditworthiness, you are able to remove the biases that are linked to it. While some agree that this approach can help relieve concerns, there are still many debates on whether the use of gender-related data should be allowed in credit lending models.
In order to protect minority groups from unjustified bias, countries have put anti-discrimination laws in place. The United States prohibits the collection and use of gender data. In the European Union, the collection of gender data is allowed, but the use of gender as a feature in the training and screening models is prohibited for individual lending decisions. However, these laws put in place to protect people inadvertently end up creating a disadvantage for machine learning (ML) driven processes. The omission of protected attributes such as gender can very well lead to worse ethical outcomes than when protected data can be used to correct for unjustified biases.
Along with researchers from the Smith School of Business at Queen's University in Canada and Union Bank of the Philippines, Aboitiz Data Innovation carried out a study – based on a use case in non-mortgage fintech lending – engaging in explainable and responsible AI efforts. We investigated whether excluding the use of gender information in assessing creditworthiness hurt rather than help the groups they are supposed to protect. Our approach tested various anti-discrimination scenarios – with and without gender-related data and measured the impact of regulatory constraints on AI model performance, gender discrimination, and firm profitability.
Ultimately, we found that regimes that prohibit the use of gender substantially increase discrimination and decrease firm profitability. On the other hand, when gender data is used, we observed an average of 285% decrease in gender discrimination and an 8% increase in firm profitability. It is also revealed that the more advanced ML models are, the less discriminatory, of better predictive quality, and the more profitable the outcomes are compared to traditional statistical models like logistic regression.
Implications and ways forward – responsible collection and use of gender data
The growing adoption of algorithmic decision-making requires us to rethink current anti-discrimination data policies that have originally been put in place for human-driven processes, specifically with respect to the collection and use of protected attributes for ML models. Our analysis points to the importance of allowing for the responsible collection and use of gender data where a win-win is achieved: the minority group is treated with greater fairness, and higher profitability is achieved for the businesses. Empowering organizations to collect protected attributes like gender would, at minimum, give them the ability to assess the potential bias in their model and, ideally, take steps to reduce it. Increased data access should therefore come with greater accountability for organizations.
This work paves the way for the fair economic welfare of both the financial institutions and the individual customers by approving loans for individuals who deserve financial support but are currently discriminated against when traditional modelling approaches or regulatory-binding guidelines are applied. The customers' chances for economic well-being are improved, and likewise, the profitability of the lending company increases for cases with lower default risk. The collection and use of gender should be supported by a strong customer communication strategy; the benefits of using personal attributes should be well described, and a suitable level of education on responsible AI should be carried out to increase customer confidence.
Banking on responsible AI to help address inequity and increase profitability
We take the concern of discrimination and the risk of unfair decisions seriously and apply methods to reduce these, especially where there are concerns with regard to AI. In this case, it is imperative for organizations to understand their lending models and modelling techniques, giving them the ability to assess biases in their models and tweak them accordingly. Leveraging AI/ML and other next-gen technologies presents organizations with a huge opportunity to help address key issues, such as inequities in the current financial system.
We need to continue making leaps and bounds in having these broader conversations and carrying out collective actions around the responsible use of AI with trustworthy partners. This way, technologically driven lending decisions will not amplify biases but instead further advance equality while ensuring that business goals are also met.