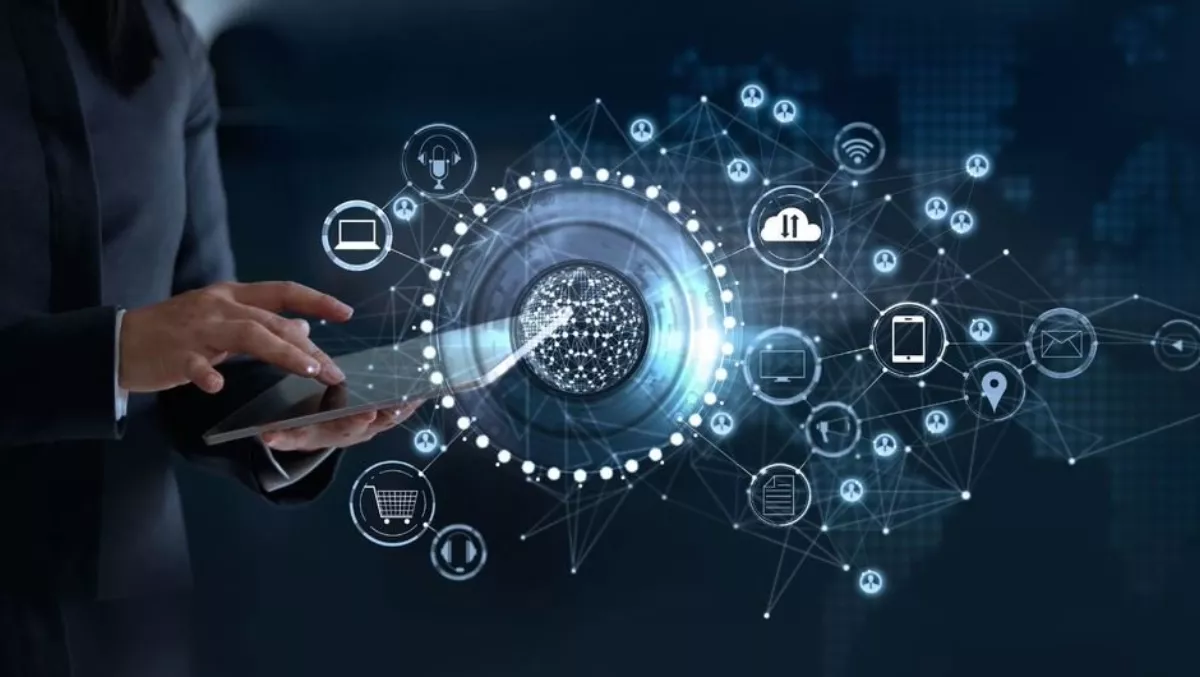
Machine learning: Moving from algorithms to enterprise-wide adoption
Although conversations about machine learning in Australian enterprises are happening, the long-term strategic application of the technology still eludes many organisations. Local deployments in artificial intelligence (AI) have utilised machine learning in just 52% of cases, according to a recent Infosys survey.
Machine learning technology will be mission-critical to unlocking new business value and measuring performance, but it's not as far up the priority list of Australian businesses as it should be.
To drive new insights and accelerate their businesses, the time is now for enterprises to get on board with machine learning. The Australian Federal Government is a great example of an organisation that understands the value of machine learning and its adoption. It has earmarked $29.9 million in the 2018 Federal budget to grow Australia's capabilities in AI and machine learning, including support for planning future investments.
Overcoming the barriers to machine learning adoption
Machine learning is ultimately about obtaining new intelligence through masses of data—so much so, that it seems organisations are now encountering a sense of data burnout. The amount of complex data that businesses are required to sift through can make the data seem overwhelming.
Recent research found, on average, Australian firms extracted 12% less value out of their data operations than firms in the rest of the world. Reasons cited include a lack of competition and a fear of failure, showing that Australian businesses aren't making use of the data obtained from machine learning, despite its growing adoption. There also seems to be a lack of understanding of what machine learning actually is and what it can do for a business—frequently amplified by the fact machine learning lacks shared vocabulary.
From different understandings of input to confusion over the name itself (AI? Deep learning?), different silos within an organisation can arrive at very different interpretations of machine learning. This lack of alignment between teams can make it difficult to inspire an organisation to look beyond an algorithm and embrace the many strategic benefits of the technology.
Got the algorithm…now what?
Machine learning uncovers new insights within vast volumes of data using algorithms. These algorithms ultimately reduce the time it takes people to spend on monotonous, labour-intensive tasks, such as anomalous behaviour detection and frees up teams to focus on creativity, product development, and improving the customer journey.
While complex, the algorithm is often the 'easy' part. Once the data has been collected using an algorithm, organisations then have to aggregate it, refine it, and adapt it to different datasets. Combine this with a lack of publicly labelled datasets, and organisations are often making a start on machine learning without any clear parameters.
Machine learning and the data it produces cannot be used to drive business value without the right people on-hand to program and train the AI. An influx of data is an often insurmountable obstacle in the machine learning journey—particularly when we consider that some 66% of the data science jobs in the Asia-Pacific Region go unfilled each year.
As machine learning is increasingly integrated into the inner workings of business operations, organisations will need to ensure they get the right employees on board who are familiar with the systems in use and how to read and interpret the data they generate. Only with the right people and processes in place will machine learning be utilised to its full potential.
Developing an effective data strategy and the right skills take time, but in the long term, this will help streamline and automate the process of collecting and extracting data, ensuring businesses are always reaching beyond the sum of the algorithm's parts.