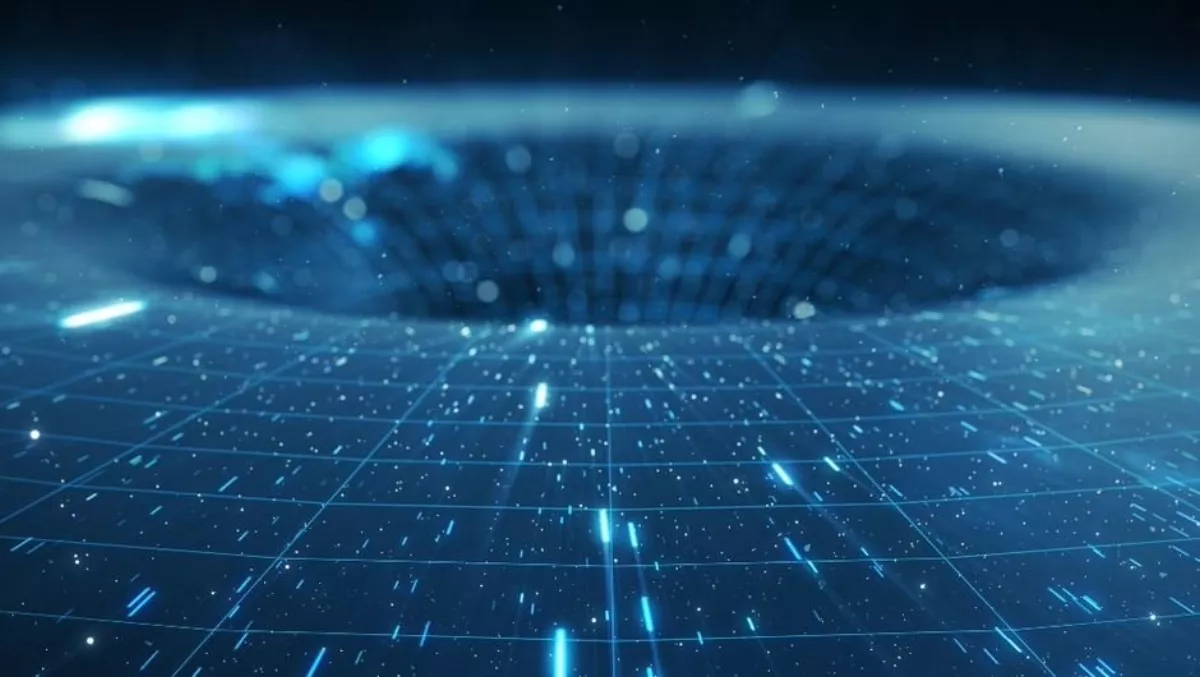
NVIDIA sets records with their enterprise AI
Backed by Google, Intel, Baidu, NVIDIA and dozens of technology leaders, the new MLPerf benchmark suite measures a wide range of deep learning workloads.
Aiming to serve as the industry's first objective AI benchmark suite, it covers such areas as computer vision, language translation, personalised recommendations and reinforcement learning tasks.
NVIDIA achieved the best performance in the six MLPerf benchmark results it submitted for.
These cover a variety of workloads and infrastructure scale – ranging from 16 GPUs on one node to up to 640 GPUs across 80 nodes.
The six categories include image classification, object instance segmentation, object detection, non-recurrent translation, recurrent translation and recommendation systems.
NVIDIA did not submit results for the seventh category for reinforcement learning, which does not yet take advantage of GPU acceleration.
A benchmark on which NVIDIA technology performed particularly well was language translation, training the Transformer neural network in just 6.2 minutes.
NVIDIA engineers achieved their results on NVIDIA DGX systems, including NVIDIA DGX-2, featuring 16 fully connected V100 Tensor Core GPUs.
Performance on complex and diverse computing workloads takes more than chipsets. NVIDIA's stack includes NVIDIA Tensor Cores, NVLink, NVSwitch, DGX systems, CUDA, cuDNN, NCCL, optimised deep learning framework containers and NVIDIA software development kits.
The software used to achieve NVIDIA's MLPerf performance are available in the latest NGC deep learning containers.
The containers include the complete software stack and the top AI frameworks, optimised by NVIDIA.
How enterprises use the containers:
- For data scientists on desktops, the containers enable research with NVIDIA TITAN RTX GPUs.
- For workgroups, the same containers run on NVIDIA DGX Station.
- For enterprises, the containers accelerate the application of AI to their data in the cloud with NVIDIA GPU-accelerated instances from Alibaba Cloud, AWS, Baidu Cloud, Google Cloud Platform, IBM Cloud, Microsoft Azure, Oracle Cloud Infrastructure and Tencent Cloud.
- For organisations building on-premise AI infrastructure, NVIDIA DGX systems and NGC-Ready systems from Atos, Cisco, Cray, Dell EMC, HP, HPE, Inspur, Lenovo, Sugon and Supermicro supposedly put AI to work.