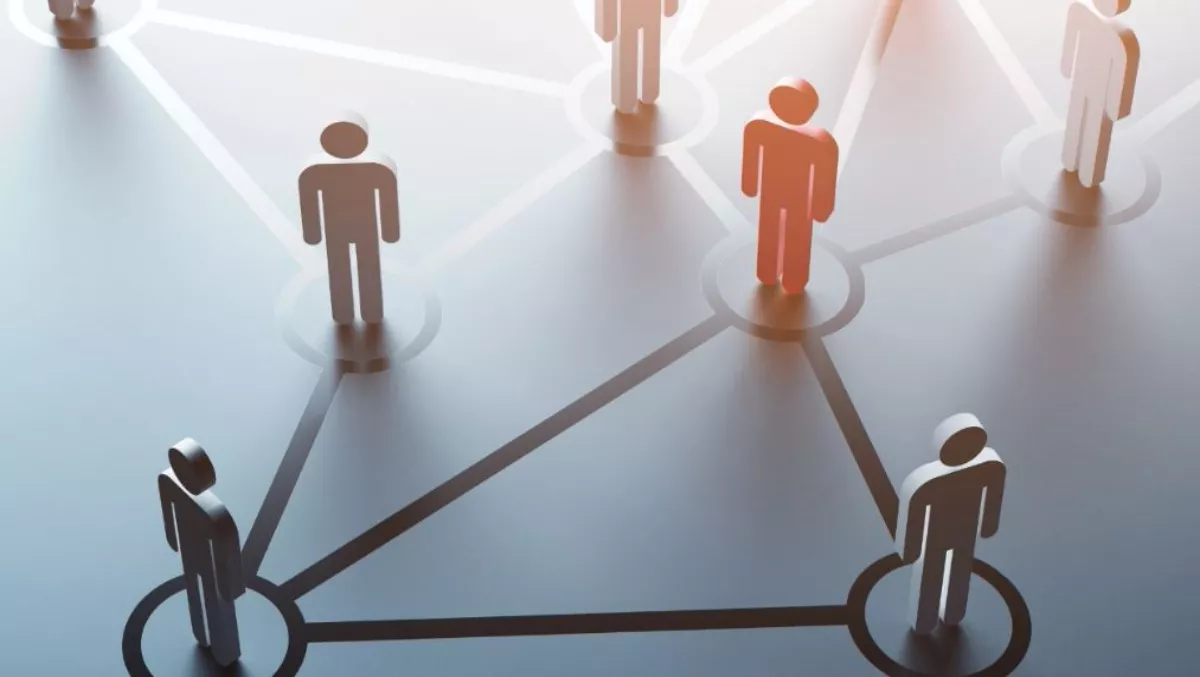
Predictive analytics: Not as hard as you’d think
Predictive analytics still sounds incredibly futuristic—and incredibly out of reach for most businesses. As a buzzword, it's most commonly attached to top-tier data analytics solutions, which reside well outside the price range of smaller businesses run by mere mortals. But the logic behind predictive analytics can benefit any organisation—and it's not as wildly futuristic as the term might make it sound.
From simple past to future perfect
Predictive analytics promises to help businesses identify a potentially important event ahead of time—in other words, to see the future. That may sound like the domain of witchcraft and wizardry, and in some cases (like certain vendors' product literature!) it is. However, it's not so hard to gauge the probability of mission-critical events occurring if you're observing the right signs in your data.
Ultimately, any predictive analytics solution revolves around a set of rules. Usually, you can break these down into some sort of “if this, then that” syntax. If storage space dips below a certain percentage, then your IT administrator receives an alert; if the temperature in said storage racks goes beyond the boiling point, then the fire department receives an alert—you get the idea.
These examples aren't predictive in nature: by the time they trigger an alert or an action, the events you're trying to avoid have already happened. But with a little bit of adjustment, they can be anticipated.
Let's take your standard storage space alert that we outlined above, which reacts to conditions like having insufficient space to perform a task. We can easily turn that alert from reactive to proactive by assessing both the percentage and actual amount of disk space left.
Five per cent free disk space may not seem like much, for example, but it's still a reasonable amount for many applications if you're talking about a 1TB or 2TB disk. And we can make that alert even smarter if we have a decent idea of how much space the business consumes every week. That's how simple predictive analytics is! You don't need fancy software or magic spells to see into IT infrastructure's future.
The magic ingredient
What you do need, however, is data—not necessarily more of it, but the right bits. Having a proper sample size of data certainly helps the accuracy of your predictions: 2 years worth of storage consumption records, for example, will help you gauge the rate of consumption better than just 2 weeks.
But more often, the strength of any predictive system depends on not the size of your data set, but its details. If you're trying to predict when your storage drives might fail, for example, collecting data from thousands of drives won't help unless you know which specific heuristics emerge just before a crash.
IT can minimise false positives, false negatives, and other predictive inaccuracies by investing time into identifying the right data.
Often, reactive monitoring provides valuable clues as to what might foreshadow a critical event. Analysing past logs and correlating different reactive alerts can build up a more comprehensive picture of the “if” conditions to look out for, and the thresholds at which your predictive analytics system should act. The “Five Whys” approach works well here: ask “why?” five times when investigating an incident, and you'll usually identify both the root cause as well as the entire chain of events that it precipitated.
This isn't just a one-off process, either. While predictive systems often start off generating a fair volume of false positives, constant refinement of their models should ideally result in more and more accurate predictions. It all comes down to how much time and effort you want to invest in your model.
Prognostication or proper value?
The question every IT leader should ask of predictive analytics is obvious: is it worth it? Do the business savings exceed the time and capital that your team invests in calibrating the system? My view is that it does—if predictive analytics is part of a broader strategy around proactive monitoring.
I've often estimated that 10 hours of investment into proactive work—any task that addresses infrastructure issues ahead of time, instead of waiting for something to happen—can save me hundreds of hours in reactive tasks if I just let things be. In general, a proactive approach to monitoring makes for far smoother day-to-day operations and far fewer business risks in the long run.
The decision to go predictive will come down to a cost-benefit analysis—and that'll differ for every business. You might measure it based on hours spent versus hours saved. Twenty hours spent writing proactive code, for example, might save the business the equivalent of an employee's full-time hours for a month: that's a worthwhile investment. Or you might translate time savings into dollar values—calculating the costs of data growth on your infrastructure, for example.
Whichever approach you take, the only real way to define the value of predictive analytics and proactive monitoring is to try them out, even in small pilots or certain areas of infrastructure. No crystal-ball gazing can beat hands-on experience in modelling your organisation's future.
By Thomas LaRock, Head Geek, SolarWinds