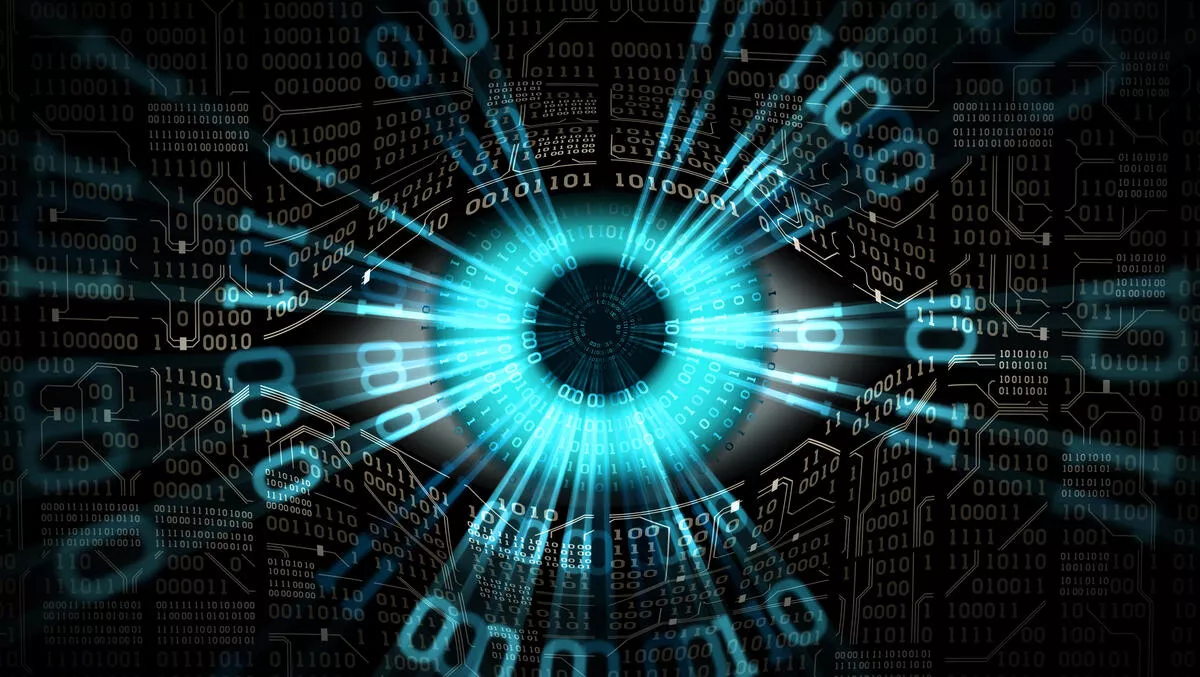
Raising well behaved AI on a global scale
AI means different things to different people and businesses. From seeking very straightforward, classical rules-based AI, to a more connectionist approach with neural nets, companies are challenged with making sure they understand the right AI to introduce with appropriate timing and scale. However, none of this will be possible if we fail to build responsible AI based on rigorous standards and guidelines.
AI is typically used to augment human abilities, from optimising company decisions to enhancing individual skills. But a number of ethical dilemmas can rear their heads while AI-based systems strive to achieve this purpose. For example, systems need to access an individual user's information, which inevitably leads to questions about data privacy.
Another challenge is that biases may exist within the vast amount of data that algorithm designers use to build AI systems, which can cause generalised and skewed results and can lead to inaccurate outcomes. There is also the possibility that people intentionally manipulate and fabricate information in a way that unfairly targets specific user groups for purposes such as racial discrimination.
As artificial intelligence (AI) systems gain widespread adoption, the need for explainability and trust in the decisions made by these systems is increasing. Explainable AI addresses the major issues that hinder our ability to fully trust AI decision-making — including bias and transparency and through its application, can help ensure better outcomes for all involved.
With these risks in mind, how do we build AI that's well-behaved?
Responsible AI is explainable AI
There is no doubt of the benefits of AI, but in seeking these new opportunities we can risk compromising the privacy of our world. A recent study by the University of Queensland, identified only 16% of Australians approve of AI, while 96% want it to be better regulated. This ambivalence suggests that widespread applications of AI in enterprise and more consumer-focused applications of AI will only progress in Australia and globally if we can raise well behaved AI that gains the trust of institutions, regulators and individuals.
The behaviour of these autonomous systems will need to be governed, unbiased, accountable, and most importantly explainable – this truly is the cornerstone of responsible AI.
But at the moment, it's becoming harder and harder to understand how AI-based systems arrive at decisions. Responsible implementation of AI and data must reflect the ethics and values of our institutions and communities. By enabling appropriate data utilisation and making AI behaviour explainable, enterprises can only then build trust among customers, employees, and other stakeholders.
Augmenting human capabilities and bias of AI – a balancing act
Ethical dangers can be addressed by creating international standards and guidelines that build solutions around fraud detection and scrutiny while bringing in human intervention where required. Building trust is also important to ensure users are comfortable sharing their data and they are aware of the processes and potential uses.
Bias in AI comes from humans who pass along their prejudices in the training data they provide, or within the models they build. A pragmatic evaluation of bias is essential to ensure the 'right set of data' is made available for AI systems to 'learn' from. Amazon had to do away with its recruiting tool that showed bias against women. In 2019 Facebook's ad serving algorithm was reported to discriminate by gender and race.
Differential privacy is a new approach that includes adding random noise into the data mix so that the resulting algorithm can be difficult to crack - even for hackers who have access to auxiliary information. But while bias detection and mitigation algorithms are available to balance the data, the best way to detect any anomaly is still through human intervention, which unfortunately cannot be scaled.
The most effective machine learning algorithms are those that can self-learn and improvise based on fresh input from real data. Once a multi-disciplinary, research-based algorithm is developed, it should be tested and re-trained based on the results to ensure bias is avoided without impacting its predictive capability.
It's very encouraging today to see enterprises looking to adopt a comprehensive approach and roadmap to scaling enterprise-grade AI for their business. There's a need for organisations to future proof and efficiently scale AI investments enterprise-wide while managing risks, this need led to Infosys applied AI, our AI offering that converges the power of AI, analytics and cloud to deliver new business solutions and perceptive experiences.
There's nothing more fundamental than intelligence. We are seeing occupations, enterprises and whole value chains changing, increasing the need to enact policies that require the responsible application of AI.
We've already seen the development of the Australian Privacy Principles come into force and make a difference on our shores. Developing standards and guidelines like these at an international scale could very well play an important role in the path to recovery of the global economy, and beyond.