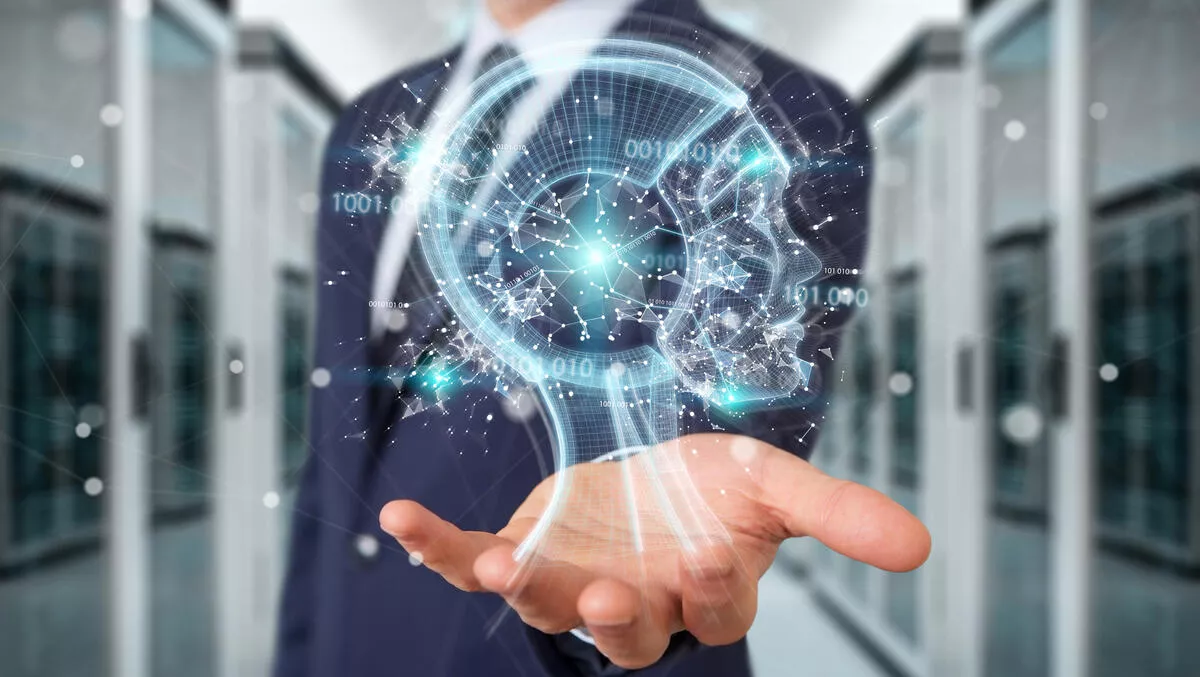
SnapLogic: How AI bias is holding back adoption
Artificial intelligence (AI) is already having a considerable impact on businesses across industries, and its influence is showing no signs of slowing down.
There are few sectors that have not yet been touched by AI.
However whilst the development and continued innovation around AI is going to bring benefits to business and our wider society, it is important that any potential risks are managed appropriately to prevent AI bias and allow AI to advance responsibly.
Research from SnapLogic found that ethical and responsible AI development is a top concern amongst IT leaders, with 94% believing that more attention needs to be paid to corporate responsibility and ethics in the application of AI.
But it is not just IT and business professionals who are concerned about AI.
As governments also look to implement AI strategies, they too are making it clear that transparency and responsibility will be a top priority.
The New South Wales government recently announced that it will be launching its AI strategy in March 2020 and pledged that transparency would be top of the agenda.
There's no question that the insights AI offers can be highly beneficial, however, we must also recognise its limits in providing perfect answers.
Data quality, security, and privacy concerns are real and until these are addressed, the AI regulation debate will continue.
What is holding AI back?
AI bias is a key hurdle that must be overcome, occurring when an algorithm delivers prejudiced results due to incorrect assumptions in the development process.
AI bias is often 'built-in' through the unconscious preferences of the humans who create the programme or select the training data.
Issues can also be found throughout the entire process, for example in the data-gathering stage, where weighting procedures can result in incorrect conclusions to be made about certain data sets.
Bias is a real concern as we become increasingly reliant on AI and we've already seen legal cases emerge where groups have forced the disclosure of how algorithmic processes make decisions.
An example of this involved teachers who were not paid performance bonuses.
They won damages when it was realised that the algorithm assessing eligibility for the bonus did not take into account class sizes – a factor found to be highly significant in pupil attainment.
Unless AI bias is addressed and eradicated, we can expect public trust of AI to remain an issue as well as potentially more legal cases as organisations and individuals seek complete transparency over how AI makes decisions.
Where does bias creep into AI processes?
The difficulty with AI bias is that it can be challenging to pinpoint exactly where it enters the system. Bias can form at any stage of the learning process and it does not always relate to training data alone – it can also emerge whilst collecting data, setting objectives, or preparing the data for training or operation.
The initial process of collecting, selecting, and cleaning data is commonly associated with AI bias.
In this early stage, bias can arise if data outliers are perceived as irrelevant and aren't thoroughly tested, allowing for prejudices to be accidentally introduced.
This can result in certain factors being mistakenly favoured by the AI, such as gender.
For example, if a successful male-dominated business uses AI to screen candidates and the AI is trained on the CVs and employment data of current employees, it is likely to develop a bias towards males.
It may disregard any female applicants for interviews as they don't fit the success pattern of the company as it currently exists.
Care must be taken when addressing this, as a simple fix like removing the sex of employees from the training data may not work.
Instead, the AI algorithm may identify patterns of male-dominated hobbies as indicators of desirable employees, for example.
Setting the objectives for a deep learning model can also be where bias forms, but those handling the process can prevent this by setting contextual objectives in order for recommendations to be accurately generated.
Finally, bias can also be introduced during the stage where data is prepared for processing.
This often results in certain attributes for the algorithms being prioritised over others.
It is imperative that this stage is completed accurately, as the choice of what attributes should be considered or ignored will have a significant impact on the accuracy of the results.
Designing a data pipeline that can handle exceptions is crucial to ensure there is sufficient data for good training outcomes.
If we don't know exactly where AI bias stems from, how can we prevent it?
IT and business decision-makers need to be aware of possible bias and how AI can be used in a way that doesn't encourage it or allow it to be accidentally introduced.
Testing is paramount – bias is often only discovered when a system goes live, by which time the issue can become far more challenging to address.
Testing the system against expectations as it develops and involving a diverse group of stakeholders in the evaluation is critical to its accuracy and success.
Progress in combating likelihood of bias
When it comes to investigating the source of bias, it is often found that human involvement, feeding into the underlying systems, is responsible for introducing prejudices.
However, once this has been identified and resolved, developers should also check the underlying data to confirm that it is fully representative of all factors that could inform the business decision. Many developments have been made in regard to this – algorithms have now been created that can effectively detect and reduce bias, which is a significant step in the right direction.
The European Union's GDPR regulation is an example of government attempts to avert the negative effects of AI bias.
GDPR gives consumers the right to an explanation of how automatic decisions have been made based on their data.
It also protects consumers as AI, and the various profiling methods it is used for, cannot be used as the sole decision-maker in choices that can have a significant impact on the rights or freedoms of individuals.
For example, AI alone cannot decide if someone is eligible for a bank loan.
Driving forward data-focused approaches
As industries look to reduce the potential risks in order for AI to advance responsibly, it is paramount that AI-enabled results reflect global, diverse data.
There are valid concerns that AI decisions often reflect biases of first-world cultures, which work against the less well off in society.
To combat this, developers need to ensure the inclusion of inputs from wider, more globalised and diverse data.
In addition, building AI models using original data can help to eliminate bias as there is greater scope for ideas and actual evidence to feed into AI systems that can, therefore, evolve to offer insights beyond the typical first-world perspective.
This data-driven approach would also build far more flexibility and responsiveness into AI systems and expose them to a more comprehensive and diversified set of global considerations.
A data-driven approach is definitely the way forward, but to allow for this, it is important that there is a focus on developing systems that break down data silos, enable seamless data integration, and ensure consistent data access and information flow.
This is possible without the need for expert software developers, as there are intuitive, self-service tools on the market that can integrate large volumes of data between different systems.
Data is key for any AI system and ultimately, there must be regulations in place that protect against bias, but also allow for continual data access and information flow.
The more decisions that are made as a result of incorrect data from prejudiced assumptions, the more challenging it will be for AI to continue to innovate and progress.
Those who invest in ensuring they can access a wide and diverse pool of data will benefit the most by identifying the true value of AI and preventing bias from influencing decisions.