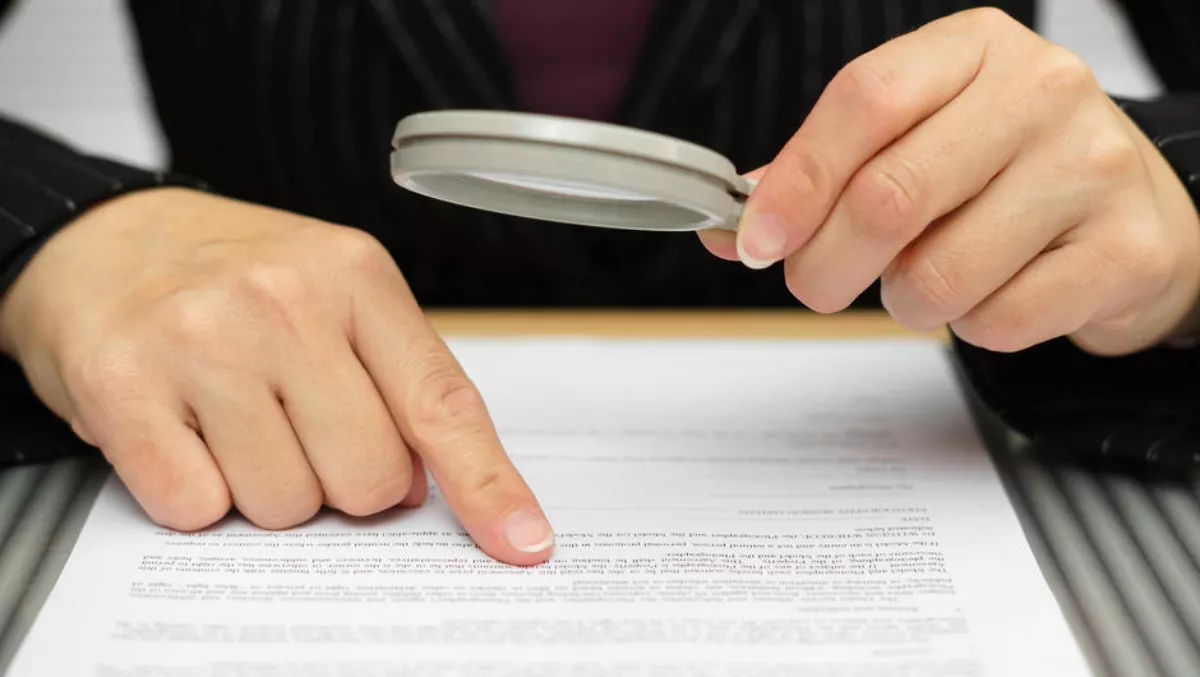
Taking a look at the growing trend of text analytics
Text analytics can help engineers who are performing sophisticated numerical analysis identify groups of ideas and concepts that can lead to better outcomes. The challenge with the process, however, is that the sheer volume of unstructured, raw text data sets can make it difficult for analytics tools to quickly and intuitively extract all the valuable information that may be available to the user.
For engineers in industries such as automotive manufacturing, aerospace design, industrial automation, and machinery, or energy distribution, choosing the right tools is an important early step to efficiently pulling insights from raw text. I'm going to explain how engineers can extract more value from raw text and combine that with sensor data and machine learning algorithms to improve functions like predictive maintenance.
What kind of text data is important to engineers and why?
One of the major areas where we see text being used is in gathering and analysing data from automotive maintenance reports. For example, these maintenance reports include information from the vehicles that can prove valuable to automotive engineers. There's text in those reports from mechanics about the vehicle's service history.
At the individual level, a maintenance record describes what happened at that particular service visit. But, if automotive engineers can quickly and easily aggregate all of those reports, then there's a lot of real-world information that can be deciphered from the correlations. For example, automotive engineers could learn the vehicle's common service issues or, from a warranty perspective, understand key failures in the car that happen simultaneously.
Topicmodeling applied to mechanics notesidentifies the key reasons for performing maintenance (Copyright: 1984–2018 The MathWorks, Inc.)
On the other hand, many of today's maintenance logs are digitised and generated automatically. In the industrial automation and machinery space, this could mean that -- during the operation of heavy equipment -- the text from these digitised maintenance logs could be analysed so that error messages or warnings are sent to operators prior to failure, thereby avoiding production having to be stopped.
Finally, Advanced Driver Assistance Systems (ADAS) in the automotive industry is a growing area for text analytics. When a car's camera captures images from road signs, those images need to be interpreted. Text analytics is a way to not only build models to read road signs but also to interpret the meaning of the text on those signs.
What other things are customers exploring when it comes to text analytics and maintenance?
Predictive maintenance is an area that could directly benefit from text analytics. We already talked about how being able to easily generate insights from raw text data from maintenance records can provide benefits; however, this raw text data can also help engineers build algorithms to predict failures before any warnings are sent. For example, in the off-highway commercial space, if a piece of heavy equipment breaks down, that becomes a costly failure.
We have customers producing heavy equipment that is going to be used on a construction site. When that piece of equipment fails, it results in more cost and time since the construction is stalled. For engineers in the industrial, automation, and machinery space, to be able to build algorithms that can predict these failures before they occur will prevent delays, thereby saving time and money.
One example of this would be to use text analytics to analyse maintenance logs and come up with categories of failures. These categories could be thought of as "labels" that identify the type of failure that occurred. By combining these labels with the raw sensor data from the equipment when it was in operation, an engineer could train a supervised machine learning model. That model could then be used on new sensor data to predict future failures.