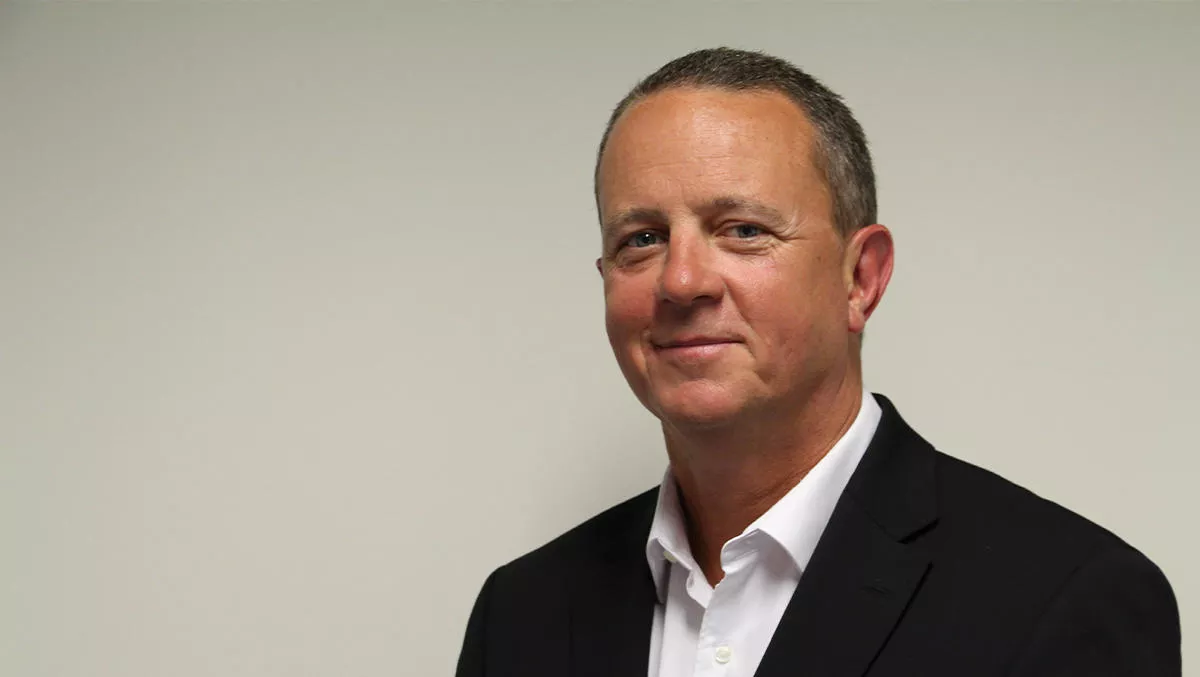
The six critical factors for a successful data science project
Keen to find ways to stay a step ahead of their competitors, growing numbers of organisations are embarking on new data science projects. Properly designed and implemented, such projects can provide valuable insights into everything from market sentiment and mood to the performance of manufacturing facilities and supply chains.
These powerful capabilities have come about due to the ongoing evolution of business intelligence, data science, and machine learning. This evolution has resulted in a noticeable shift in focus from descriptive analytics to predictive analytics during the past few years. Rather than studying what has already happened, it's now more about forecasting what is going to happen.
Yet, despite the significant value that data science can deliver, there are still some significant hurdles that need to be overcome. Indeed, according to Gartner, 85% of big data projects fail to move past their preliminary stages. The company also predicts that 80% of analytics insights will not deliver business outcomes before the end of 2022.
A key reason is that many of the tools and processes being used within projects are still firmly rooted in academia and science. They require deep technical knowledge and rely on complex manual processes to work.
At the same time, the data within organisations needing to be analysed tends to be still very siloed. It is often stored in multiple locations and without any easy way for the data within them to be accessed and combined.
A third key barrier is the challenge of attracting and retaining sufficient people with the required data science skills. Competition is tough, and the number of people with these skills remains relatively constrained.
Factors for success
To overcome these challenges, organisations can take six key steps to maximise the success of data science projects. These steps are:
Provide fast and easy access to data
One of the most critical factors in any data science project is having readily available access to the required data. To achieve this, begin by getting a clear picture of the existing data infrastructure and how it may need to be evolved. Ensure sufficient resources are dedicated to data management and realise that this will be an ongoing task.
One option is to take advantage of the Data Cloud. This approach can decouple compute resources from storage resources and ensure that a single copy of the data is readily available to anyone who requires it.
Provide secure access to third-party data
Using internal data stores is important, but so is having reliable access to needed external sources such as partners, vendors, suppliers, and even clients.
The fast and efficient exchange of data with these groups can be critical to completing sales and transactions, monitoring supply chains, and gaining post-sale insights into how an organisation's products or services are being viewed in the market.
Having efficient external links in place also means data can be readily pulled into analytics tools to generate accurate insights as quickly as possible.
Build efficient data pipelines
Once effective access to data has been achieved, the next step is to build pipelines to deliver it to all teams involved in the project. These could be teams focused on BI development, analytics, or machine learning.
It's important to have data pipelines that are as standard as possible so they can serve multiple groups of users. This will remove the need for the constant development of pipelines for specific, one-off use cases.
Choose the right tools
As data science has matured, there has been an increasing number of tools entering the market. Some have succeeded in adding value, while others have quickly disappeared without a trace.
When selecting tools, organisations should choose those that most closely match their particular business use case. Determine the outputs that are required and work backwards to select and evaluate the most appropriate tools.
Embed data science into the business
It can be tempting to focus on the technologies and tools associated with data science. Yet, one of the most important factors to consider is how this data science is embedded within an organisation.
For this to happen, there must be executive sponsorship for the data science program as it needs to be seen as an integrated initiative that drives value across the entire organisation. To achieve this, data scientists should work closely with senior managers to develop a strategy that balances technology with business goals.
Build a data science team
Hiring the right people is critical for the success of any new data science initiative. Interestingly, the best prospects are likely to share a set of similar traits. Firstly, they are likely to be naturally curious and always asking 'why?'.
They will also be good communicators who can explain business challenges using data. The best will also be team players who are happy to share experience, insights, and successes with others.
By following these steps, the likelihood that a data science project will succeed will be significantly higher. Taking time today to plan, evaluate, and manage data, tools, and people will ensure real business value is achieved.