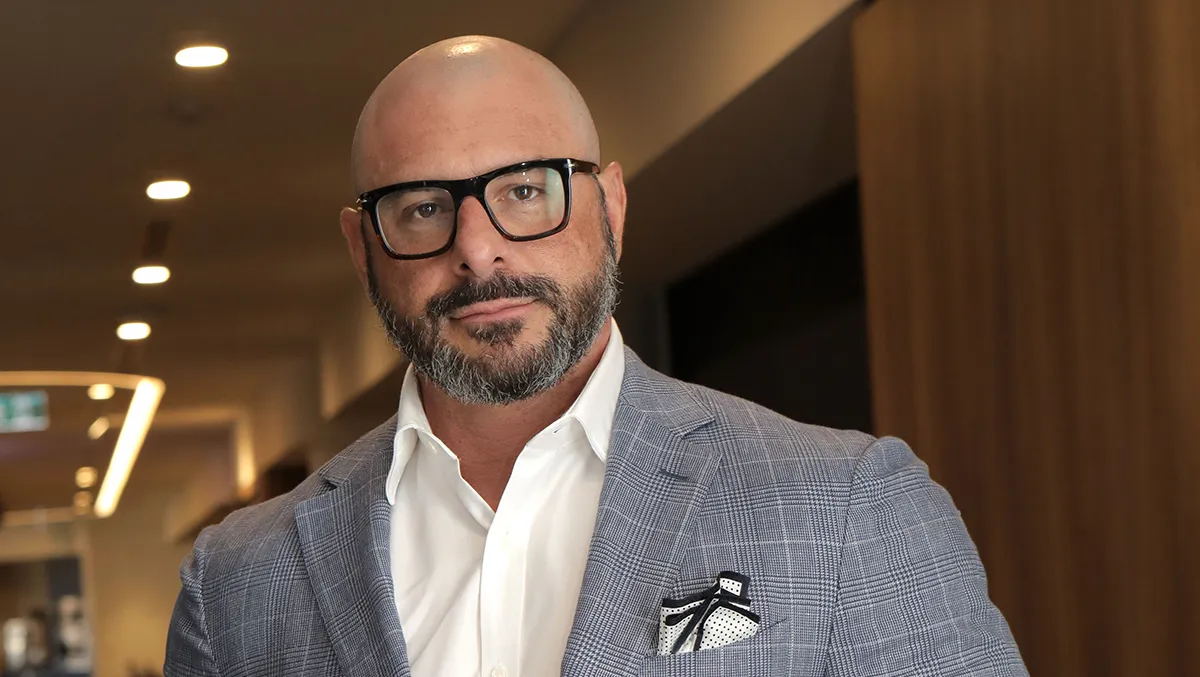
Over half of all data held by organisations is ‘dark’ and unused, but it would be a brave call to suggest it holds no value - it may be more valuable than the data currently feeding analytics experiments.
Australian organisations are good at capturing and storing data from across their operations; where they typically run into issues is determining how much of that data is valuable and able to be distilled into actionable insights.
A big part of the problem is thinking small.
In data analytics, organisations often set up small-scale experiments, tackling a large dataset by carving out what they see as a representative sample, cleansing it and running it through advanced analytics algorithms in the hope of determining what latent value that data holds. That value can then be further unlocked by productionising the use case.
However, large datasets are complex. What looks like a representative sample to experiment with may not be. In turn, what gets extrapolated from a small subset of data is not always representative of what would be extrapolated from analysing petabytes of the same dataset.
And so Australian organisations may be short-changing themselves: artificially limiting the value they can derive from data based on overly small-scale experiments and - more critically - making important decisions based on the results of constrained experiments.
What is needed is some more expansive thinking on advanced analytics.
Experimentation today should be powered by more data, if not the whole dataset, then as much of it as possible. That means cleansing and preparing as much of the data as possible, not cherry-picking a small chunk of it. Unconstraining this early stage by having more clean data to feed an analytics model is important to recognising and unlocking value.
For many organisations, that will mean having to develop new capabilities or mature their existing capabilities to classify, cleanse and convert data into a format where they can look at it, understand what it’s telling them and what picture it’s painting, and make specific decisions based on it.
Artificial Intelligence (AI) technology is emerging here as a useful aid, reducing the amount of human intervention required to filter, sort and catalogue this plethora of data and helping organisations to reach accurate judgments on the value of their data holdings much faster.
Judging data’s real value
Data is important to a wide-variety of organisational decision-making today.
The obvious use is in business intelligence-type decisions to drive commercial outcomes, particularly in functional areas of the organisation, such as influencing the direction of new product or service development and enhancement or formulating business development and sales growth campaigns.
Here, it makes sense to know that decisions are being made on the most accurate reading of the underlying data since the margin for error can be material in terms of cost or timing. The last thing an organisation wants is to get data-driven decision-making wrong because not enough data from the core dataset was utilised.
But it’s not just commercial decisions and leaders’ judgement that may be impaired when too little data is used to drive actionable insight.
Small-scale experiments can also lead organisations to incorrectly identify on an ongoing basis the data they need to keep collecting to keep generating the same actionable insights. This can lead to incorrect decisions being taken, either by data owners or by IT teams whose infrastructure is used to store and process that data, to stop collecting or storing certain types of data or data fields altogether or to permanently ignore - or even delete - corresponding historical data.
The obvious repercussion there is that data that has value is lost because a small-scale experiment did not have access to it, so it could not deem it useful - and that this decision gets reinforced by further incorrect decisions.
We saw evidence of this in research this year that found the vast majority of Australian companies (69%) store every piece of data they can just in case, but 51% of the data is ‘dark,’ or never used. It is a big call to say that half of all data being collected holds no value, yet that’s the way it is being treated.
Another emerging role for AI
As we move into 2024, the message to Australian organisations is twofold.
First, to open their minds and broaden the scope of data experiments to utilise more data. That might mean classifying, cleansing and converting more of that data into an easily ingestible format upfront, but at least then, a correct call can be made on what data is and isn’t useful for producing actionable insights and powering data-driven decision-making efforts.
Second, organisations should start to explore the use of emerging AI technologies that can separate the wood from the trees, or in this case, the useful data from that which is redundant, obsolete or trivial (ROT). AI has an increasing role to play in reducing the human effort that is required to ensure clean and relevant data is at hand, reducing the risk of valuable data going dark and to waste.