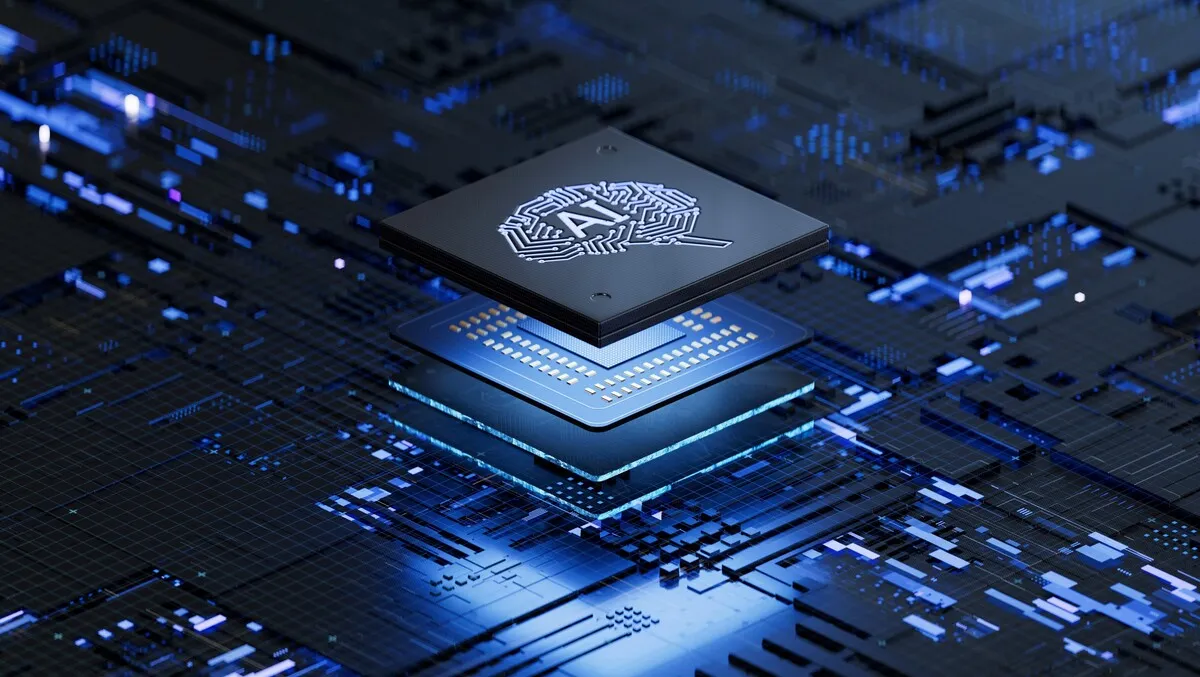
Accelerating AI Innovation Needs Ecosystems and Infrastructure
The advent of ChatGPT has taken generative AI mainstream, and many organisations are focusing on accelerating their AI initiatives to better serve customers, employees and partners. All organisational functions, such as sales, marketing, finance, support, operations, IT and product development, are looking to use AI to streamline and improve their internal workflows.
The question is: Will they have the necessary skill sets, systems and infrastructure in place to handle the massive disruption that AI will have on their operating models? Creating scalable AI solutions requires businesses to accommodate the ingestion, sharing, storage and processing of enormous and diverse data sets while keeping sustainability in mind. We refer to it as production-grade AI.
In the Equinix 2023 Global Tech Trends Survey (GTTS), we learned that 42% of IT leaders believe their existing IT infrastructure is not fully prepared to accommodate growing AI adoption. Also, 41% doubt their team's ability to implement the technology. In Australia, respondents were more optimistic (35% and 36%, respectively). Participating in digital ecosystems and choosing the right technology partners can be instrumental in helping organisations deploy the right infrastructure in the right places when they need it most. In effect, your ecosystem becomes your infrastructure.
Production-grade AI deployment introduces new challenges
IT teams are beginning to support the use of AI technologies across their organisations but face an entirely new set of challenges around cost, performance, data sharing, skills gaps and sustainability.
Predictable cost models
Organisations have the following cost-related concerns around AI:
- By the middle of this decade, the majority of data will be generated outside the data centre. The cost to backhaul data generated at the edge and sent to the core can be prohibitive. If the data is generated in the cloud, it makes sense to process the data in the cloud. However, if the data is generated at the edge, it should be stored and processed at the edge. Thus, centralised AI architectures will not scale with respect to cost and performance.
- Enterprises also want a predictable fixed-cost model for their AI Infrastructure. With a fixed cost model, businesses will know in advance how much it will cost for their AI infrastructure every fiscal quarter. That is, the cost does not vary based on the number of developers or the number of workloads or jobs. Furthermore, clouds have variable costs, such as data access costs and egress costs. There's a charge for every data request to the storage and for moving data out (egress) of the cloud. Depending upon the workload, these variable costs can become a larger percentage of the overall storage cost.
Optimising AI performance
Organisations are encountering barriers to high performance for the following reasons:
- Access to the latest GPU technology: AI training jobs take less time with newer GPU technology, but it's getting increasingly difficult to access the latest GPU technology in the cloud. Working with an older generation of AI technology increases the cost of customer AI training runs.
- Inference latency/throughput: When data is generated at the edge, moving this data to a centralised location for AI inference increases response latency.
- Variance in the system and deployment architecture: Even if GPU vendors, OEMs and clouds all use the same type of GPUs, there will be a difference in the overall performance of these deployments due to GPU interconnect architecture to networks, storage and other GPUs in the cluster. This difference in performance applies whether the AI system is deployed on dedicated infrastructure or shared infrastructure, and whether there is a layer of virtualisation or if the system is running on bare metal.
Data sharing challenges
In many cases, organisations need to leverage external data (e.g., weather data, traffic data, etc.) to improve the accuracy of their AI models. For most AI projects, one does not build an AI model from scratch. Instead, one uses an external AI model as a starting point and subsequently customises that model with their private data. Thus, organisations need to know about the lineage of the external data and models they are using to ensure they are not violating any compliance regulations and to protect themselves from corrupt data that malicious agents have manipulated. This will be especially true once people start leveraging open-source-based foundation models.
Similarly, many organisations want to monetise their data with external parties. However, these data providers want to have control over the data they plan to share, to prevent unauthorised use cases or forwarding this data to non-paying actors. Unless these data sharing challenges are overcome, this will inhibit the use of AI in enterprise environments.
Skills shortage
Most organisations are finding it difficult to hire qualified AI workers. In the GTTS, 45% of IT leaders reported their biggest skills challenge is the speed at which the tech industry is transforming. Businesses need enterprise architects knowledgeable about emerging AI hardware and software architectures, data scientists, data engineers and data curators to work on AI projects. Generative AI solutions are, in many cases helping to bring AI technology to the subject matter experts and the end users in a seamless manner. Furthermore, many Software as a Service companies provide enterprises with solutions incorporating AI features.
Enabling sustainability/green AI
Organisations recognise the need to do AI sustainably and want to do their part. Increasingly, organisations must show the carbon footprint of their IT infrastructure to customers, employees and partners. The GTTS reported that less than half of IT decision-makers (47%) are confident their business can meet customer demand for more sustainable practices.
AI training racks consume greater than 30kVA per rack, and air cooling becomes inefficient; higher kVAs per rack require liquid cooling. Most private (in-house) data centres are not equipped to handle these power-hungry AI racks. The increased demand for transparency by stakeholders has also raised concerns by organisations over the water usage effectiveness (WUE) and power usage effectiveness (PUE) of the data centres hosting their IT infrastructure. Stakeholders will also likely want to know what portion of the IT infrastructure is powered by renewable sources.
Is your infrastructure ready for AI?
Running high-performing distributed AI infrastructure on Platform Equinix helps IT infrastructure teams overcome AI complexity and manage massive data volumes, freeing up business units to start realising the tremendous value of AI solutions. Participating in digital ecosystems gives you access to new technology partners with innovative solutions that will help solve production-grade AI issues and fast-forward your company's AI strategies for competitive advantage.