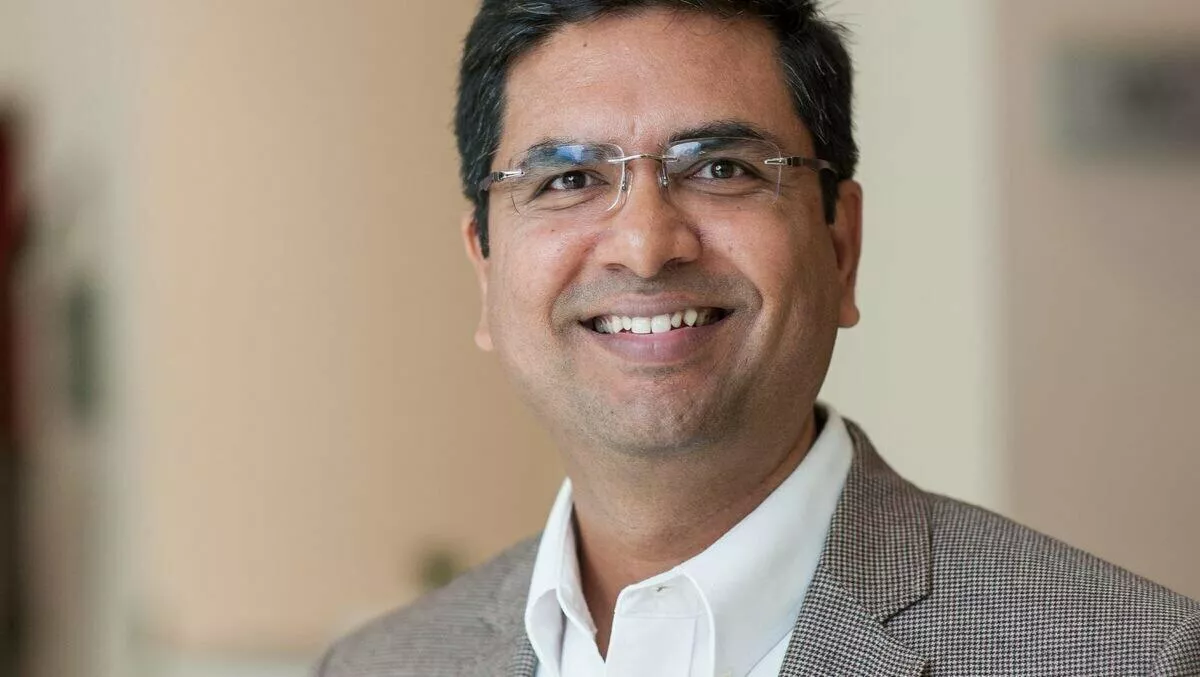
Data integrity key to a secure, AI-driven enterprise - ManageEngine
If artificial intelligence (AI) hasn't formed part of the conversation in your enterprise yet, then you're behind the eight ball.
Many organisations in the Asia Pacific region have their cheque books open and are embracing AI.
According to IDC, annual spending on cognitive and AI systems in Asia Pacific countries, excluding Japan, is expected to reach $15.06 billion in 2022.
AI will provide opportunities for Australian organisations to innovate and differentiate themselves across all areas of business, according to PwC.
Some sectors will see wholesale business model transformations while others will find localised opportunities to deploy machine learning.
While AI technology is rapidly transforming business and industry, organisations looking to leverage it must ensure the integrity of the data that powers their systems by addressing four key issues: safely collecting and storing data, preventing concept drift, handling adversarial attacks, and stamping out human bias.
Safely collecting and storing data
Data and data analytics are at the heart of many AI projects, and the integrity and security of the customer and company information used to inform AI systems are critical.
Successful use of AI's enormous potential requires systems that can collect and store significant volumes of data safely.
However, business data and AI models often contain sensitive information, including personal data belonging to customers and employees, which makes them a target for cybercriminals.
Implementing multiple layers of security like encryption and continuous monitoring, particularly while data is in use within AI models, may be necessary to ensure data isn't stolen or compromised.
It is essential to treat this set of data the same way as other sensitive data; building multiple layers of security, including encryption, accomplishes this.
Where encrypting data can hinder AI systems, organisations should look at technologies like homomorphic encryption, the method for performing calculations on encrypted information without decrypting it first, to balance between AI system benefits and information security.
Preventing concept drift
AI models are only as good as the data that powers them.
The phenomenon of concept drift, where the intelligence acquired in the AI model based on operational data suddenly changes drastically, results in the data models becoming irrelevant.
Predictions or actions based on these models may result in security issues, such as data theft, exposure, or deletion.
Concept drift could also be engineered by intentionally supplying wrong data to business applications, as typically the AI systems have no intelligence to ascertain if they are learning from the right or wrong data source.
Updating the learning models periodically is one remedy.
This approach is best suited for machine learning algorithms that use regression algorithms and neural networks, both of which fall under the umbrella of AI.
Handling adversarial attacks
In addition to securing the data storage infrastructure, organisations must be aware of the quirks with the AI learning process that can be exploited by attackers.
Most prominent of them is the adversarial attack designed to intentionally fool the AI system by supplying it with wrong data that results in the system learning the wrong things and applying it to its eventual behaviour.
Two methods have proven to be a successful defence.
Adversarial training provides a brute-force solution by generating numerous adversarial examples and training the model not be fooled by them.
Defensive distillation utilises probabilities based on data provided in an earlier model but which has been evaluated and modified so that it is difficult for hackers to find points of attack to exploit.
Stamping out human bias
It's reasonable to assume that the predictions and responses generated by an AI-powered system would be unbiased.
However, that's not always the case.
Human bias can be engineered into AI systems if skewed or non-inclusive data is fed into the business applications that AI models are based on.
Once this has been accomplished, attackers may be able to control the functioning of the business application to generate non-objective outcomes in their own favour.
Working with a strong initial data set is vital for attaining good results from AI, and minimising the potential for bias.
The biggest roadblock for enterprises to combat bias is the data silos that often accompany rapid cloud adoption.
Automation and integration technologies that create cohesive workflows can ease this problem, and enable businesses to keep their data clean and error-free, so it works seamlessly with AI.
Why explainable AI is a powerful protection measure
One definitive way for organisations to avoid the four key issues mentioned above is to invest in "explainable AI."
Explainable AI offers reasoning for why the AI system arrived at various predictions and provides explanations for actions it would like to carry out before executing them.
This introduces the opportunity for people to counter engineered factors like concept drift, adversarial attacks, and intentionally planted bias in real-time.
Ensuring data isn't stolen or compromised is vital to a successful rollout of AI technologies.
This can be supported by implementing multiple layers of security that utilises homomorphic encryption, continuous monitoring, and other strategies.
A well-informed organisation with well-meaning leadership will understand the nuances of all such concepts, and the need to invest in a holistic system that fully leverages the benefits of AI technologies.
Implementing AI-driven systems is fast becoming a requirement for Australian businesses trying to stay competitive in the marketplace.
Those who don't will find themselves struggling to compete.
AI-driven systems can deliver significant benefits to organisations willing to invest in them.
And making security integral to AI initiatives means companies can enjoy the advantages without opening their systems and operations up to additional risk in the process.