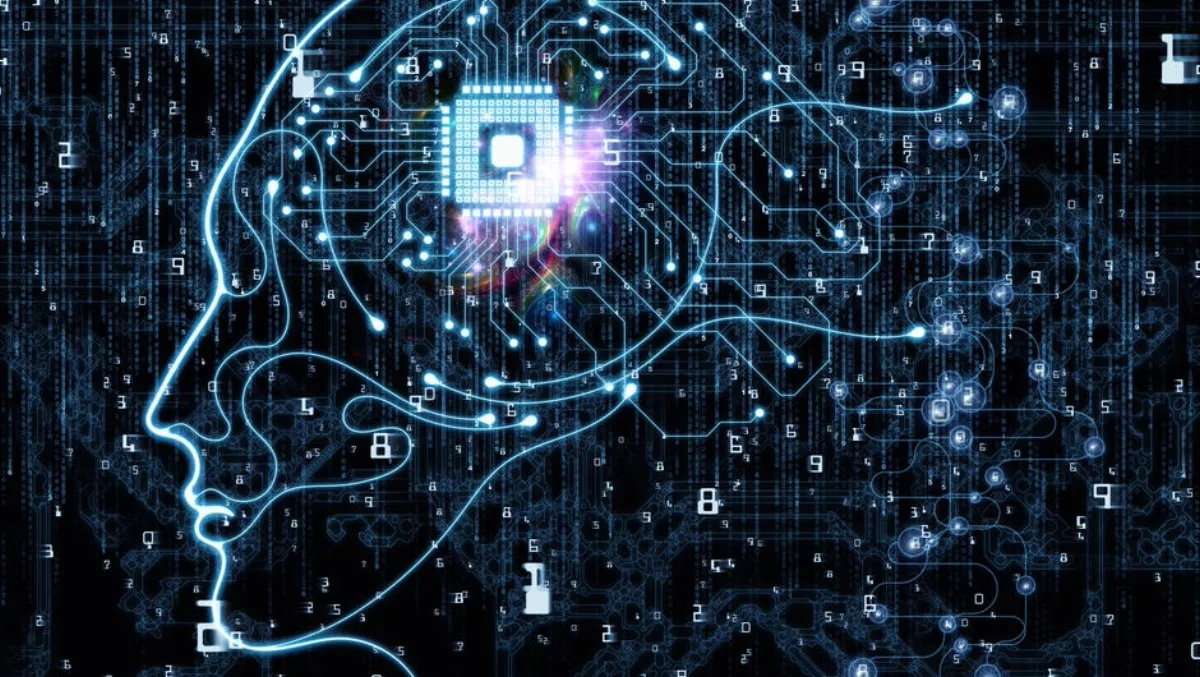
Exclusive interview: Microsoft on whether AI is worth the hype
One of the most talked about trends in the entire technology and business sector is the evolution and adoption of artificial intelligence and machine learning.
Vendors in just about every market are putting out AI and ML technologies that are facilitating a great deal of business innovation, with organisations often rushing to implement the latest and greatest solutions.
Although with the wealth of offerings that existing within the market, it might be difficult for organisations to understand where they should be investing, and whether the hype around AI is even justified.
Raghu Ramakrishnan, Microsoft CTO for data and AI has a decorated and long-standing history in working with database systems and AI.
We sat down with Ramakrishnan to find out the best ways that organisations need to be thinking about AI and, in fact, whether AI is actually worth the hype.
How has Microsoft's approach to AI evolved since the very beginning?AI has been a core investment of Microsoft for as long as I can remember. If you were to look at some of the very basic, foundational breakthroughs in deep learning, Microsoft - through its early work in speech translation - produced some of those early deep learning results and now more recently with the CNTK algorithm. There's a lot of ongoing development, with so many areas that are core to the company.
Machine learning (ML) cross fertilises so well with a plethora of different areas of the company. It fits in nicely into our data platform because ultimately ML is working from the data that is stored in databases. We've learnt from that process as well, and we've now made it possible for people to run their ML algorithms right within the database, that way they don't have to take the data out, which is a problematic process.
On the one side we have various parts of the company that produce ML algorithms and on the other side, we have the data platform teams which build data platforms with hooks to run these algorithms natively right inside. All in all, it's a nice synergistic loop.
AI is somewhat exploding in both business implementations and general interest lately. Do you think the hype is justified?It's a fair question and I can answer it with equal honesty with both a yes and a no. In most end-to-end AI applications, the truth of the matter is, your ML is only as good as the data that you give it learn from. So gathering that data in the first place is a tonne of effort and cleaning it and making sure it's not noisy is also quite difficult.
Then, there's an art to it, where you look at certain parts of the data and isolate features of it that might be more useful for an algorithm to learn from. If you try it, you can see how effective the algorithm is and then you iterate. That whole process is very intensive in terms of the queries, analysis and compute that is required around your data.
Once you do all of this, you have an instance of ML that you're using in production, but you keep repeating the process as your data evolves. Then you have to worry about things like securing your data as well as all the challenges that come from having high business value or sensitive data, including things like compliance and governance.
So if I took all of that, I'd say that there is too much excitement, because 90 - 95% of the actual work is around things to do with data. However, on the other hand, I'd say no you're not overhyping it, because in many important applications that last mile of bringing predictive learning to the table allows you to do something with that data that you could not have done otherwise. In that sense, it's a sufficient improvement to justify the investment.
Do you think organisations are going 'all in' in AI when perhaps their current systems aren't yet ready? Is there a problem with being too hasty?I'm sure that there is some of that. Although ultimately the right thing is to think about how to make your organisation data driven. Using machine learning or AI is a part of that, it's a particularly effective way to be data-driven.
You really have to look at what companies have historically been doing for the last 10 or 20 years. They have been putting their data in a standard business cube and people in the company use that as a source to determine things such as sales performance in terms of location, vertical or specific product. They look at that and they make adjustments to their business procedure to achieve what they want, which ultimately makes them data-driven. There's no AI attached to that process, but it's about gathering all the right information to make decisions about the next steps.
AI brings another exciting tool in how you use that data and if you think of it in that frame, then you solve the problem of being too hasty with the technology. The hard problem is finding the right data to focus on, understanding it, securing it and learning how to manage it. If you use an approach that represents an evolution of how you are viewing your data and how you're making your organisation data-driven, life is good.
However, if you approach it in a simplistic, purely inquisitive way - wanting to basically just come in and 'do cool things' with AI, then you're probably not sophisticated enough to understand all the nuances of being data-driven. You're also not being realistic in your expectations of what you can actually do, which will ultimately result having a poor experience overall.
Another complex environment that might be tough to navigate for organisations is IoT technologies, how have you seen that technology develop?IoT is actually one of the things that I'm personally excited about. Looking at the journey of data and analytics, for many years a large database was considered to be a few gigabytes, and then when terabyte storage came into the market, it was considered huge.
That scale remained constant for 10 or 20 years, before it was completely reset when web companies started needing to analyse web logs, which were so much bigger than anything we'd seen before. So systems like MapReduce and Hadoop were built to cater for that, and the numbers jumped up by a factor of 10 or more, which continued for a while.
I think IoT is going to represent the next big jump, as it has the potential to change industry after industry. If you look at virtually every modern building, factory and vehicle there are sensors attached to them which facilitate innovative strategic business practices. There is no industry that is not data-driven, it's not just half a dozen web companies. The volumes of data are up by an order of magnitude beyond the web companies and the number of companies has exploded.