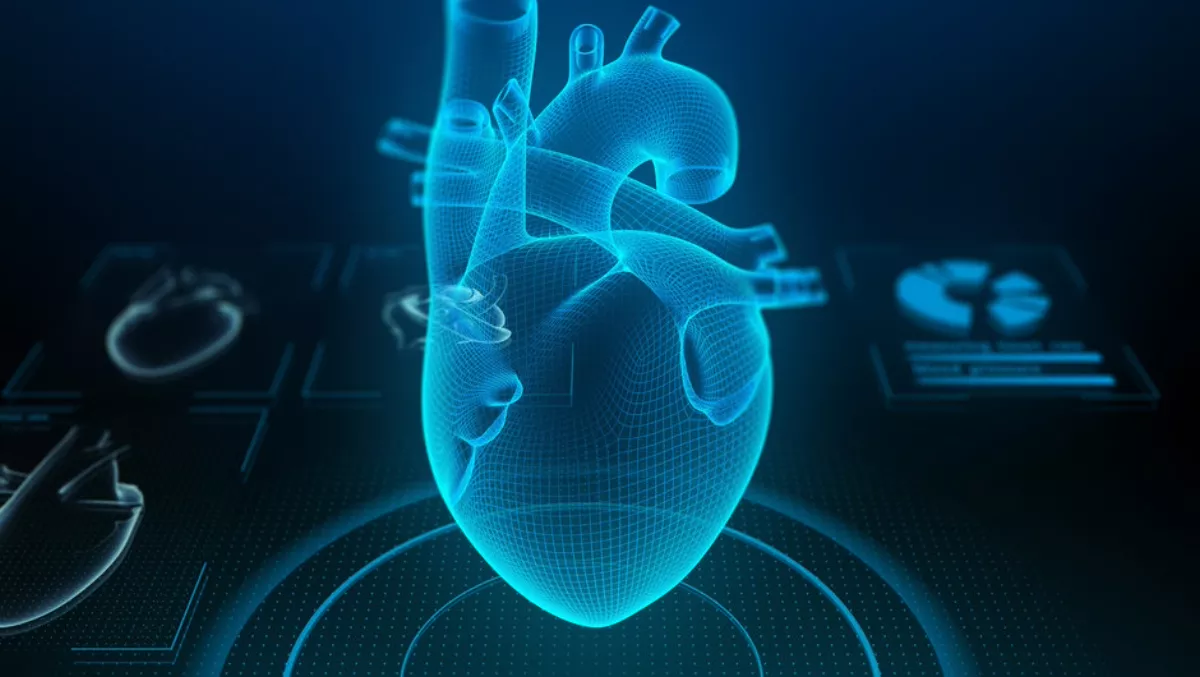
How AI could help cardiologists detect heart defects
Nearly a third of physicians will be sued at least once in their careers, most commonly for an error in diagnosis.
Deep learning has the potential to help doctors cut down on diagnostic errors, said cardiologist Rima Arnaout in a talk at the GPU Technology Conference.
An assistant professor of medicine at the University of California, San Francisco, Arnaout is focusing on the potential of AI to analyse cardiac ultrasounds and detect congenital heart disease from fetal ultrasounds.
"In medicine, a picture is worth more than a thousand words," she said. "It really can be worth a patient's life, in some cases.
What can AI do to help?
Arnaout outlined a few key challenges for humans analysing medical images. For one, people sometimes make mistakes. There's also a physical limit to how much data cardiac imaging specialists like cardiologists and radiologists can analyse.
"We cannot allow those kinds of shortcomings," she said. "We need accuracy, precision, and we need it delivered at scale.
While AI models are not without their limitations, Arnaout said, they can help clinicians use medical imaging techniques like ultrasound to their full potential.
She turned to echocardiogram data because "it's balanced in terms of information richness and clinical volume compared to other cardiovascular imaging tools." Since echocardiograms can be used for the diagnosis and management of almost every cardiovascular disease, she said, there's very little selection bias in the datasets.
Echocardiograms are a challenging training dataset, however, because one ultrasound study consists of still images and videos captured from over a dozen angles. A study Arnaout's team published in npj Digital Medicine used deep learning to classify 15 of these standard views, achieving 91.7 per cent accuracy on low-resolution images.
Detecting congenital heart disease in utero
Recent work by Arnaout focused on the detection of congenital heart disease from fetal ultrasounds. While in theory more than 90 per cent of complex congenital heart disease cases can be diagnosed through traditional fetal screening ultrasounds, the actual detection rate is under 50 per cent.
This gap occurs in part because fetal hearts are small and fast beating. Since the fetus itself is often moving, diagnostic-quality images can be difficult to obtain. And although it's the most common birth defect, congenital heart disease affects just one per cent of live births. Since it's so rare, the condition can easily go overlooked by human readers.
That's where an algorithm can help: once trained, it could reliably catch congenital heart disease in perpetuity.
Using hundreds of fetal echocardiograms from 18 to 24 weeks of gestational age, the UCSF researchers developed convolutional neural networks to distinguish two varieties of congenital heart disease. The deep learning model, trained on NVIDIA GPUs hosted on Amazon Web Services, was able to classify the two kinds of defects at well above the average diagnostic rate.
Catching heart defects early can lead to better outcomes for patients after birth. And if certain types of lesions are spotted in a fetal ultrasound, doctors can recommend in-utero therapies that significantly improve the heart's condition by birth.