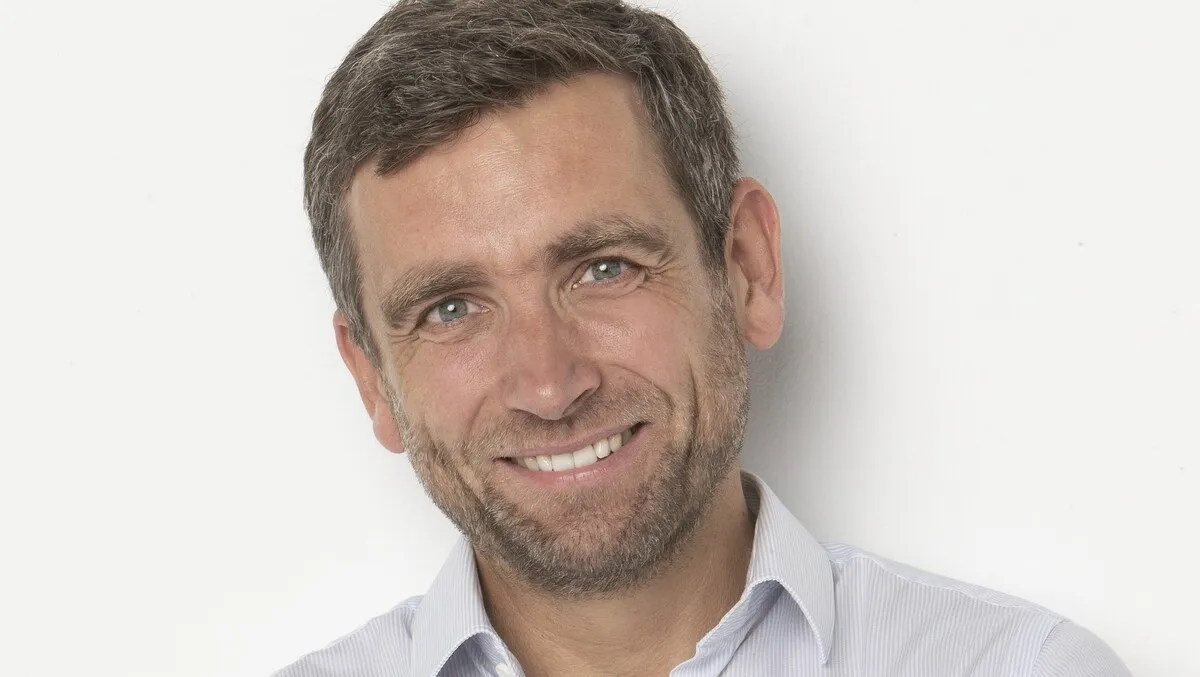
How Generative AI Will Help Businesses Improve Data Quality
When ChatGPT was released in late 2022, it captured attention worldwide. People were amazed by the platform's ability to answer almost any question (still sometimes with some failures) and generate content from news articles to poetry.
What has since become clear is that Generative AI like ChatGPT could also have an important role to play when it comes to data quality. While the use of artificial intelligence (AI) tools in data processing has tended to focus on predictive analytics, which is now shifting to natural language processing, data analysis and automation.
Fast forward to 2023, and the extent of AI use has grown exponentially. Indeed, according to the Data and Analytics Leadership Annual Executive Survey for 2023, 80.5% of data executives indicate that AI and machine learning (ML) will be an area of increased data and analytics investment during 2023. For 16.3% of them, it will be their highest investment priority.
This focus is because data quality has become critical for effective business management. It ensures that organisations can make informed decisions based on accurate, complete, and consistent information. Poor data quality can result in inaccuracies during decision-making, financial losses, and harm to a brand's image and standing.
Improving data quality with generative AI
This makes Generative AI components compelling for data analysts and why significant investments are planned. The result will be a different way of managing and analysing data, delivering even more substantial business benefits.
According to analyst firm Gartner, by 2025, at least half of all data management tasks will be automated. Most of this automation will be accomplished using AI/ML-driven tools, specifically generative language models.
This shift has significant implications for data quality. These technologies offer the potential to revolutionise data management by automating and simplifying tasks like never before. This advancement promises to improve accuracy, completeness, and consistency in organisations' data handling processes.
As an example, improvements could be achieved by following a two-step process. First, a technical data quality assessment would be conducted using ML algorithms that can identify anomalies and quantify the severity of the issues.
Following this, based on the assessment results, a generative language model would be used to suggest data quality rules and transformations in natural language that business stakeholders can readily understand.
AI-generated rules and prompts
Generative AI can also assist businesses in automating data review and creating recommended rules and prompts based on the results.
For example, a business running an online store could use an AI tool to check whether order dates are within an acceptable range and provided customer details are correct.
Business managers could also create additional rules simply by asking in natural language, without needing to write code or develop complex UIs. Once the new rules have been approved, they can be automatically converted into executable code such as Python or SQL.
Before deploying the new code to production, it must be tested and validated using a sample of data to ensure the rules are working as expected. Once the data cleansing process is completed, the refined data can be employed for a wide range of downstream tasks, encompassing data analysis, visualisation, machine learning, and business intelligence.
Data transformation
The pace of change in data management shows no sign of slowing. Although the use of generative language models such as ChatGPT is still in its infancy, we expect usage to increase.
As more businesses understand the value this new generation of tools can deliver, they will push implementation to the top of their to-do lists. But even if the promises are exciting, the technology is still relatively new, and to avoid distorted results in AI models, it's essential to be aware of this and prepared with internal training, responsible practices, or facilitating interactions with UX improvements. You'll reap massive benefits by preparing and training your organisations and teams. And one of the most significant benefits will be greatly improved data quality. Soon.