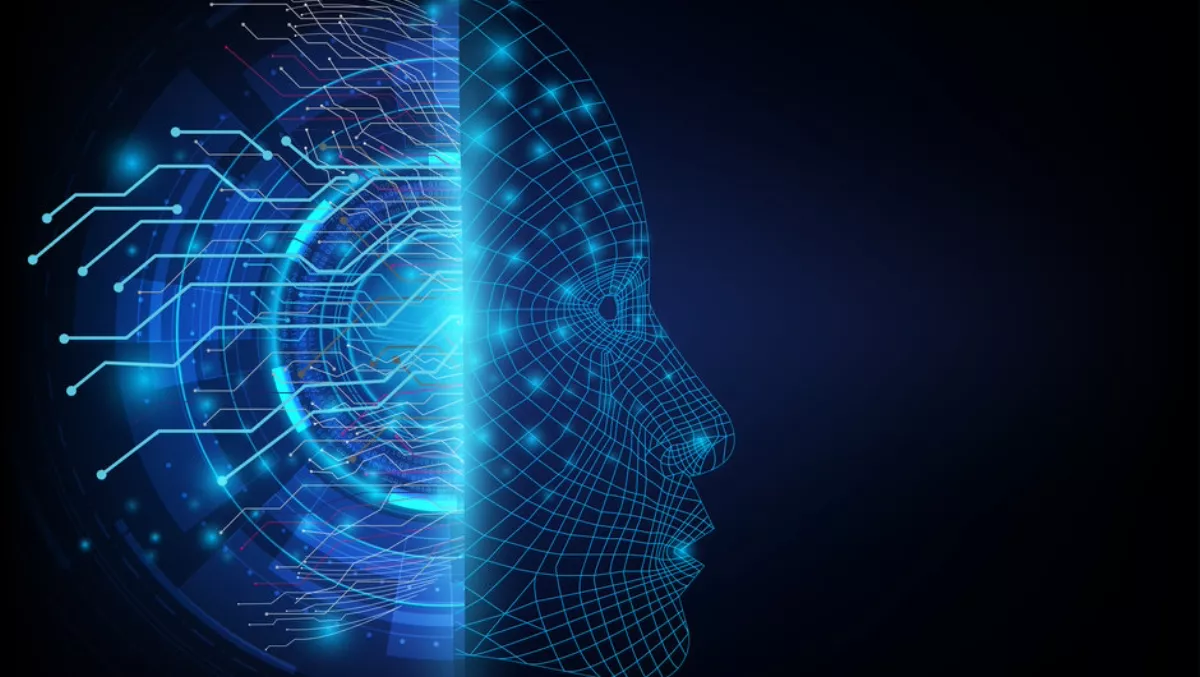
How to maximise the value of machine learning in cybersecurity
Machine learning (ML), a subset of artificial intelligence (AI), offers transformative potential in the cybersecurity sector. ML-based technologies can help fight large-scale fraud, evaluate and optimise business processes, improve testing procedures, and develop new solutions to existing problems. Like most innovations, however, ML has drawbacks, so organisations need to understand how ML can help, and how to overcome its imperfections, according to ESET.
Cybercriminals and malware developers are increasingly relying on ML tools. This is because ML can power new malware strains, target specific victims and extract valuable data, hunt for zero-day vulnerabilities, and help cybercriminals protect their own infrastructure, such as botnets.
And, increasingly, ML solutions deployed by legitimate organisations are also becoming attractive targets for cybercriminals. By creating poisoned data sets, attackers can try to manipulate these otherwise-beneficial systems into incorrect decisions, or force them to provide a distorted view of the monitored environment, causing damage, chaos and disruption for an organisation.
ESET senior research fellow Nick FitzGerald says, "It's difficult to say which effects of ML, positive or negative, will prevail. However, ML-powered systems are growing rapidly on both sides of the cybersecurity barricade, irreversibly transforming the safety of the entire internet. Without diminishing the value of ML as a tool in the fight against cybercrime, there are limitations that need to be considered.
ESET has identified four key limitations of ML in cybersecurity:
1. Training sets must be extensive: A vast number and range of correctly labelled input samples are needed, divided into three categories: malicious; clean, and potentially unsafe/unwanted. Building effective training sets can be impractical for many organisations, so it's important to work with a security partner.
However, while organisations like ESET work with millions of samples collected over more than 30 years of malware research, this is no guarantee of perfection. Subsequently, human expertise and verification are required constantly.
2. Math can't solve everything: A purely mathematical approach fails to take into account the unknown elements that can arise. The algorithm, therefore, would need to take an overprotective stance and block even safe traffic, or it would have to let potentially-dangerous traffic through.
One approach creates a huge number of false positives, while the other makes effective business operations practically impossible.
3. Intelligent adversaries adapt: Attackers will break the rules and change the playing field without warning, so it's not possible to create a protective solution today that will detect and block all future threats.
4. False positives can be destructive: For some businesses, a false positive (FP) can be more destructive than a malware infection. If an FP causes a security solution to block or delete a manufacturing line's software, for example, it will disrupt production.
This could cause massive delays and cost millions of dollars in financial and even reputational damage. In non-manufacturing organisations, FPs can translate into higher costs, overburdening of IT security staff, and even harmful adjustments in cybersecurity posture.
It's important to remember that ML can be used to create more effective malware, too. In 2003, the Swizzor Trojan horse used automation to repack the malware once-a-minute, serving each victim a polymorphically-modified variant of the malware. This required advanced detection mechanisms that detected, blocked, and mitigated emerging threats, including attacks using ML, in response.
Nick FitzGerald said, "Organisations must make ML a part of their security posture to avoid falling victim to ML-powered attacks. Attackers can use an algorithm that makes it easier to learn the limits of the implemented security solution, helping attackers alter the malware's code just enough to evade detection.
"However, the limitations of ML show that ML alone isn't enough. Therefore, a safer and more balanced approach to enterprise cybersecurity is to deploy a multi-layered solution that can leverage the power and potential of ML but backs it up with other detection and prevention technologies as well as human expertise.
"ESET's security tools have relied on ML for many years and have been proven to help reduce the number and severity of successful cyber attacks for customers. Its full potential is yet to be unlocked; organisations that aren't yet exploring the use of ML in cybersecurity should begin doing so to avoid being left behind.