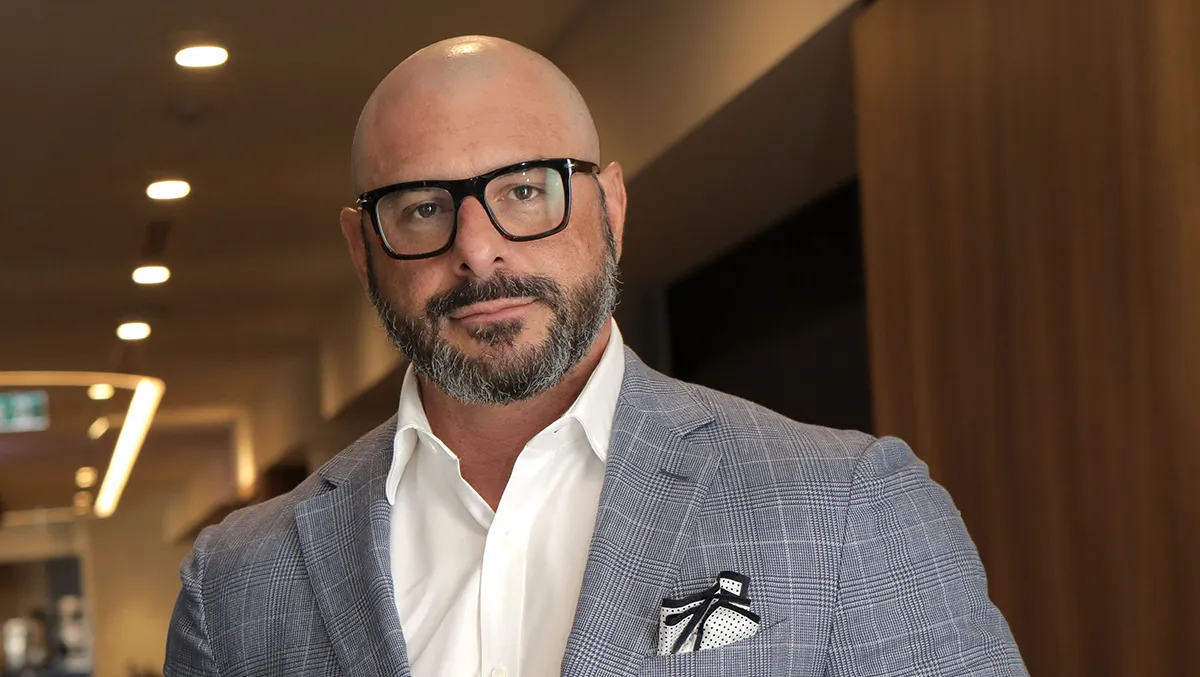
Object Storage: A balm for what ails enterprise data management
Large organisations such as financial institutions are dealing with more unstructured data than ever. What does that mean in practical terms? At a time when organisations are striving to make data-driven solutions and provide relevant, personalised services to improve the customer experience, they are only able to use a fraction of their data.
The latest research says only 2.5% of data is analysed. What's happening with the rest? Where is it, what is it, and how much of it is useful? How can it be stored and used in a meaningful way that integrates with the company's applications, cloud solutions, as-a-service solutions and on-premise systems?
It's a complex issue that impacts an organisation's ability to change and innovate, minimise risk, modernise digital offerings and anticipate customer needs.
Throw in the threat of ransomware and cyber security risks, implementing the latest phase of Open Banking and ensuring compliance with Consumer Data Right legislation, and it is clear that legacy thinking and technologies will not provide the answers.
The technology that will provide data visibility, handle increasing storage requirements and store data in such a way as to make it useful was invented decades ago: Object Storage. And the modern version of it is fast becoming a crucial part of the IT stack.
Fin Sector Playing Catch-up with Data
Over the past few months, we've been speaking with groups of professionals from financial institutions and discussing common data challenges. The sheer amount of data they are dealing with and the fact it's steadily increasing has been called "scary".
They talk of issues with legacy infrastructure and how difficult it is to act with agility. Newer fintechs haven't yet built up massive transaction histories on which to base customer personalisation and digital services decisions. Older institutions have more dark data (unclassified, unidentified data) than they can comprehend.
Their data scientists and analysts spend too much time finding, accessing and integrating data and not enough time thinking about and analysing that data. This slows down their ability to work through use cases that can provide value to the business.
They talked about dedicating data and analytics to things like compliance, retention and fighting fraud - which are critical but so challenging and time-consuming that there aren't a lot of time and resources left over to focus on finding a competitive edge.
What if an organisation could make much more of its data useful and highly accessible? Not only is the technology available with which to do just that, but it can also be done whilst maintaining legacy application stacks, modernising hybrid cloud, multi-cloud, and private cloud environments and building new, cloud native applications.
What does a can of beans have to do with data?
Imagine a storeroom filled with unlabelled cans. You have no idea what is in them until you open them. That's dark data. Dark data is data sitting in one or more storage repositories that may or may not have any value or serve any purpose to the business. The business doesn't know what or where it is or whether it is useful or not because no mechanism has been put in place to classify it and determine its value. It hasn't been deleted, "just in case." It has simply been stored.
Whatever is in the cans could be delicious, or it could be poisonous. Who knows how long it's been there or whether it has spoiled. Toxic data is data that should not be stored or should be deleted after a certain time frame because it could be a liability or security risk. For example, identity data associated with an outdated and completed contract, or contained in a report that is no longer required for compliance purposes, is toxic. Yet it is stored or retained for too long because no system has been put in place to identify whether it has any risk associated with it or to alert the business when it should be destroyed.
Now imagine that the cans have one word on them: Beans. Now you know what's in the cans. Sort of. The word bean means different things to different people. What type of beans? What flavour is the sauce, if any? Is the same kind of beans in every can? What are the ingredients? What preservatives have been used? Plus, you still don't know how long they have been there and whether they are still okay to eat. That's akin to unstructured data. Without a label telling you the ingredients, there is no structured information with which to understand what's in the cans of beans.
Unstructured data is really any data that does not conform to a defined or organised data model or structure, which makes it difficult to search and analyse. Some popular examples of unstructured data include emails, text files, photos, videos, audio files, webpages, presentations, multimedia, call centre transcripts/recordings, financial statements, claims documents, CAD/CAM, medical imaging, and the list goes on.
To apply this metaphor to financial institutions, you'd have to imagine warehouse upon warehouse of cans of beans, with more large shipments arriving every day. It would not be possible for people to keep up with opening and identifying all those cans of data efficiently, allowing for the identification, testing and usage of all those beans. Similarly, there aren't enough person hours to sift through all the information an enterprise holds to allow it to figure out what to do with the remaining 97.5% of its data.
Applying Object Storage to this metaphor would be like storing each can of beans along with all of its label data and making that information searchable and protected. Not only that, but it would also capture all the relevant data about the object, such as when it was created, when it was stored, who put it there, who accessed it and when, what changes were made to it and when etc. Plus, the cans would be compressed to enable more to be stored in the existing space, but each can's contents would remain easily and speedily accessible.
Object Storage is a revelation
Raw data comes from many sources in many formats and is stored as ones and zeros: the language of machines. To make that data useful for people and businesses, it must be transformed into information, appropriately classified and stored accordingly.
Context indexing and metadata provide information with which to understand your data. The data can now be classified, and appropriate privileges and policies can be assigned to meet retention, protection and security requirements. This process will allow the business to identify and deal appropriately with dark, toxic and unstructured data to remove associated risks. A single distributed index architecture across all data environments will ensure the business has a single source of truth.
We're seeing how deployments of object storage can reduce total costs and distribute data geographically for high availability – all the while demonstrating its ability to scale to billions of objects. It's moved past the typical backup and archive use cases and is more than ready for high-performance workloads, crunching huge data sets for AI, ML, and advanced analytics.
Data is a significant strategic asset that can yield actionable insights, and yet so much of it is crying out to be properly managed, governed and analysed. With object storage, an organisation can categorise and understand every bit of data that gets sucked into an object store. That's transformative.