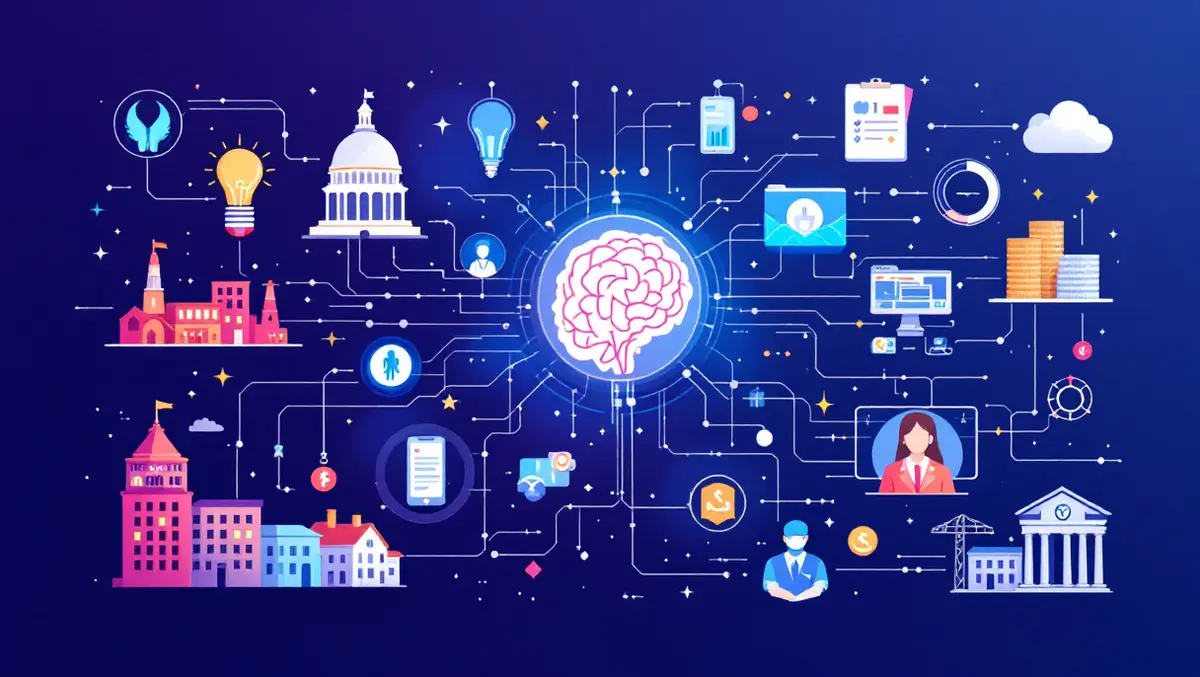
SAS takes measured path with AI to deliver real-world gains
While much of Silicon Valley pushes a vision of AI that borders on science fiction, analytics veteran SAS is choosing a more grounded path — one focused on real outcomes, not marketing hype.
AI & Models
"We're building a lot of powerful AI, but we're leaving the LLMs to others," said Udo Sglavo, Vice President of Applied AI and Modelling at SAS. "That's not our game. We use large language models like any other software system, but we're bringing them together with our decades of expertise in forecasting, optimisation and statistical modelling."
That approach is delivering tangible benefits. Around the world, SAS has worked with financial institutions and government agencies to help detect fraud, one of several early use cases for its proprietary AI models.
"These are areas where we see the biggest payback," Sglavo said. "Being able to detect fraud before it happens brings huge business value."
At its core, SAS is building sophisticated systems that combine pre-trained large language models with traditional tools to complete tasks with business context. Unlike purely generative systems, SAS agents aim for reliability and specificity. This more structured approach is essential in the finance, healthcare and manufacturing industries.
"If our team were building agents solely on large language models, I'd be very nervous," Sglavo explained. "You ask the same question twice and get two different answers. That doesn't work for critical systems."
Unlike competitors pushing rapid AI transformation, SAS takes a more incremental and customer-driven path. In addition to its own models, it works with frontier models from Microsoft, Google and many other providers. Essentially, they're vendor-agnostic in this part of their software stack. Sglavo noted that while Microsoft is a key partner, "that doesn't mean we're tied to them. We work with whoever our customers work with."
In addition to the fraud detection examples often sited, SAS has begun deploying AI for worker safety in real manufacturing environments. "Every incident is expensive, and making workplaces safer is a clear return on investment," Sglavo said.
Data, Data & Data
To reduce the risk of hallucination (where AI generates incorrect or misleading information), SAS uses a retrieval-augmented generation (RAG) architecture, feeding agents company-specific data rather than letting them scrape the open internet. "You're basically saying, go to this knowledge base, not the whole web," he said.
This flexible approach to AI extends to how SAS works with client data. One area seeing increasing interest is synthetic data — artificial datasets that mimic real-world information without exposing sensitive details. "We're getting a lot of demand for this," Bryan Harris, SAS's CTO said. "We even acquired a company, Hazy, to bolster our capabilities in differential privacy."
"Think about approving or denying a mortgage," said Harris. "You need broad, representative data to avoid bias. But many companies only have their own narrow data sets. With synthetic data, we can expand the training set safely and create better models."
Synthetic data allows SAS to model rare events without relying on ethically fraught real-world data. "In worker safety, for instance, you don't want to wait for fatal accidents to collect enough data," Sglavo said. "Using simulation, we can train models that are highly sensitive to risk without putting anyone in harm's way."
Human disruption
Harris echoed this pragmatic & flexible approach and emphasised the need for humans in the loop. "These systems are great for natural language interaction, but they don't have a value system. They're probability machines. Good human judgment remains the most critical skill in the C-suite."
"I don't believe data scientists will be replaced," Sglavo said. "It's like driving a car. Sure, your car is smarter now, but you still need to drive it. The role changes, but it doesn't disappear."
For junior data scientists and future talent, that shift means education must evolve. "It's not about learning to code anymore," Sglavo said. It's about critical thinking, understanding what tools can do, when to use them, and the ethical questions involved."
Harris agrees. "AI is going to commoditise coding in many ways, but you still need people to judge the quality of what it generates," he said. "We're seeing productivity gains from generative coding, especially in routine UI work, but maintenance and debugging remain human-led."
The SAS way
Internally, SAS is also preparing for disruption by disrupting itself. "We've built small teams whose job is to challenge what we already do," Harris said. "Show me how to do it better and cheaper. If it works, we adopt it. If not, we move on."
In a market flooded with overpromises, SAS is consciously staying measured. "We're not going to sprinkle AI on everything just to meet the hype," Harris said.
As AI adoption matures, Harris expects more businesses to value real-world results over buzzwords. "The enduring quality here is that human interaction with data — through natural language — is now expected," he said.
In the meantime, SAS is staying focused on outcomes. "We're combining decades of trusted modelling with new tools," Sglavo said. "That's what sets us apart."