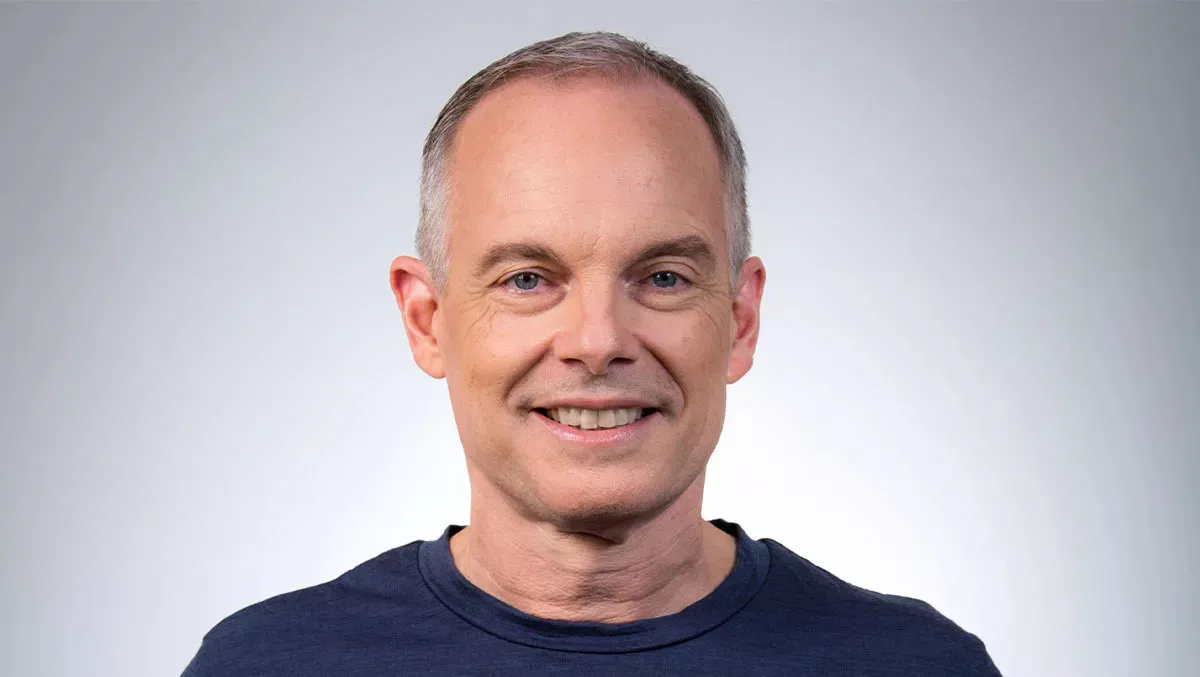
Three steps to creating a data-driven enterprise
One of the biggest challenges facing organisations today is how to truly harness the power of data - to determine what and how to measure, understand what the data actually "means," and prioritise what data to look at or act on to achieve maximum impact for the business.
A large part of the effort associated with digital transformation revolves around bringing data together to create a clear, top-level view of all data being ingested and ensure that these resources sit at the core of how a business operates.
At the highest level, there are three principles that underpin successful digital transformations through the creation of a data-driven enterprise.
Adopt a cross-functional, data-centric approach
By definition, the entire organisation needs to participate in a coordinated and coherent process to become a data-driven organisation. That means linking data from customer experience, business teams, finance, product, engineering and operations together in a way that allows alignment and optimisation to take place.
Creating that framework is a material competitive advantage for companies undergoing digital transformation. According to the McKinsey - Company study on "Ingredients for a successful Digital Transformation", the top success factor (that separated winners from losers by almost a 3:1 ratio) was "Implementation of digital tools to facilitate analysis of complex information". The next two factors were:
- Adapted business processes to enable rapid prototyping and testing with customers
- Created networks of cross-functional teams with end-to-end accountability
Businesses face several key issues when it comes to the implementation of their vision. The first is the sheer volume of possible data to be monitored.
The second is the selection of tools needed to manipulate the data across different time-horizons (real-time, in the next few days, this month, this quarter, this year, year-on-year) and for different stakeholders (business, product, marketing, engineering, eCommerce, etc.) and the economics associated with acquiring and running the data tools themselves. Making this even more challenging is the shortage of available data science/engineering professionals.
As a result, fragmentation of information across disparate, incompatible tools is an issue for most companies out there. Ironically, quantifying the impact of this fragmentation is difficult to impossible, given there are very few organisations (if any) that have extensively rolled out observability across the entire organisation to allow them to quantify the impact.
Enable collaboration across the enterprise
Collaboration is not just about SREs, operations personnel or developers working together. It's about how these functions collaborate with and support product management, growth teams and finance teams to work as a cohesive unit. This cohesivity enables scalability and the ability to manage more complex environments.
Many organisations don't have a clear view of their data. A common reason is that they don't have a central/integrated data management platform. It is also common to find several similar data stores existing in different parts of the company and no systems that provide a holistic view across these sources.
Another problem arises when organisations make investments in data tools but cannot integrate or normalise data across these tools sufficiently, which eventuates in creating multiple versions of "the truth" and the requirement to constantly try to determine which data to follow. Timeliness is also a major issue: if it takes two weeks to get the data needed today, then, of course, it won't be very helpful.
In all these cases, it's difficult to make data-substantiated decisions if an organisation doesn't have the data or isn't confident in it. In addition to being suboptimal from a business efficiency standpoint, the less visibility one has, the more likely this will translate into performance and customer experience problems.
While achieving a "single pane of glass" view is more of an optimistic goal, what we're looking for is the equivalent of a diamond: where all of the information is holistically related, and different stakeholders can view it through their "facet" (e.g. their function or related KPI, OKR or dashboard).
The key to achieving all of the above is to have a strong culture that first and foremost understands the value of a data-centric organisation - which includes observability - but is extended to the organisation as a whole. These cultures will invest in data and observability and will constantly be looking to evolve to that "multi-faceted diamond". Their proportional investments in data (and observability) reflect this culture, and not surprisingly, they are better able than their peers to manage their business.
Implement AI, ML to handle scaled complexity
From an observability standpoint, the main job of Artificial Intelligence (AI) and Machine learning (ML) is to help us humans deal with the exponential explosion of data. The sheer volume of data available for analysis, along with the number of possible relationships between the different data sources and points, makes it impossible for humans to sift through and react to the data in real-time.
AI and ML are good at doing several things with this sea of data: pattern recognition, correlation and predictability (in a probabilistic sense) - and can achieve this analysis at speeds and volumes several orders of magnitude greater than humans can.
Through correlation, AI and ML can quickly detect relationships that would not be easily detectable otherwise and bring these relationships to the attention of either humans or other systems. This includes detecting patterns that commonly occur before an issue takes place, allowing the team to investigate and determine if the anomaly is benign or an early sign of an impending issue that should be addressed before it impacts the customer or the business.
One well-known example of these ML capabilities is Google's AI arm DeepMind. Despite the tech giant's data centers already being highly optimised by some of the best engineers on the planet, DeepMind algorithms identified additional optimisations that reduced cooling costs by a further 40 per cent.
Even more savings (or reduction in additional costs) will be achieved from a resource perspective due to these algorithms minimising the need to materially scale teams as data volume scales. However, the ultimate value is customer experience and satisfaction as AI and ML reduce downtime and increase performance.
Although tech teams can now automate much more than they once could with these new AI and ML capabilities, there probably won't be a complete handover to automation any time soon. Instead, organisations should look at the current state of AI and ML in observability to keep up with the volume and complexity while minimising the need to scale the teams responsible for observability.
To get near that state of "total automation", a much broader percentage of an organisation's data will need to be incorporated in the observability framework. In addition, more sophisticated ways of helping the algorithms learn will need to be implemented.
By New Relic APJ chief technology officer, Michael Fleshman.