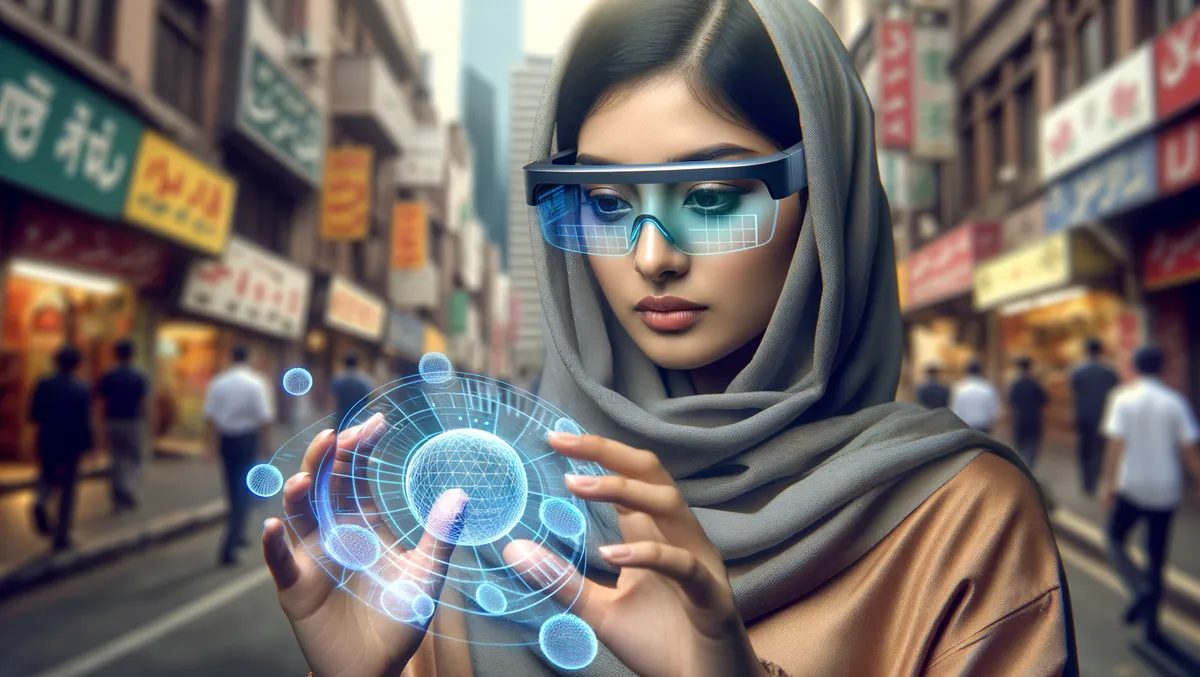
WiMi develops new AR system using DCNN for dynamic image recognition
WiMi Hologram Cloud, a global Hologram Augmented Reality (AR) Technology provider, has announced the development of an innovative augmented reality (AR) system capable of recognising and tracking objects in real-time in dynamic scenes.
Defined by its adaptation of deep convolutional neural network (DCNN) algorithms for image recognition, the system leverages DCNN's significant capacity for feature extraction and classification in order to improve recognition accuracy.
The deep convolutional neural network (DCNN) structure is primarily utilised for tasks within the realm of image recognition and computer vision. The core mechanism behind DCNN's success in image classification and recognition lies in its ability to learn image features through multiple convolutional, pooling, and fully connected layers; each layer is composed of a certain number of neurons.
DCNN provides an accurate standard for object recognition by tapping into different levels of image features and methodically extracting more abstract characteristics.
The most significant component of DCNN, the convolutional layer, extracts features via a convolutional kernel which performs operations on the input image. This process involves application of the convolution kernel to obtain an output feature map. By doing this, DCNN learns low level to high level features and consequently extracts more abstract features.
Additionally, DCNN utilises a pooling layer designed to retain critical feature information while reducing both the feature map size and the number of parameters. The final fully connected layer of the DCNN uses neurons to classify the outputs into one-dimensional vectors.
The adoption of the DCNN as the base model by WiMi has allowed for a streamlined processing and superior recognition of dynamic imagery. The technology has been adapted to accommodate the processing of dynamic images and enable efficient information transfer and tracking between frames in quick succession.
The recognised objects are combined with augmented reality to create real-time augmented reality effects. This integration of virtual objects with real scenes offers users a more immersive and interactive experience.
The DCNN-based augmented reality dynamic image recognition offers vast potential for adoption in sectors such as gaming, education and healthcare, providing a richer, more immersive augmented reality experience for users.
Its applications are varied, from the recognition of dynamic characters in game development and identifying vehicles in intelligent transportation systems, to identifying equipment and products on production lines in industrial settings. The fusion of deep learning and augmented reality enhances the efficacy and accuracy of dynamic image recognition methods.
WiMi emphasises the technology's potential for further development and enhancement, communicating its intention to strive for performance advancement and broadened application scope. Planned improvements are based on model optimisation, dataset expansion, and multi-modal integration to better support applications in the field of augmented reality.